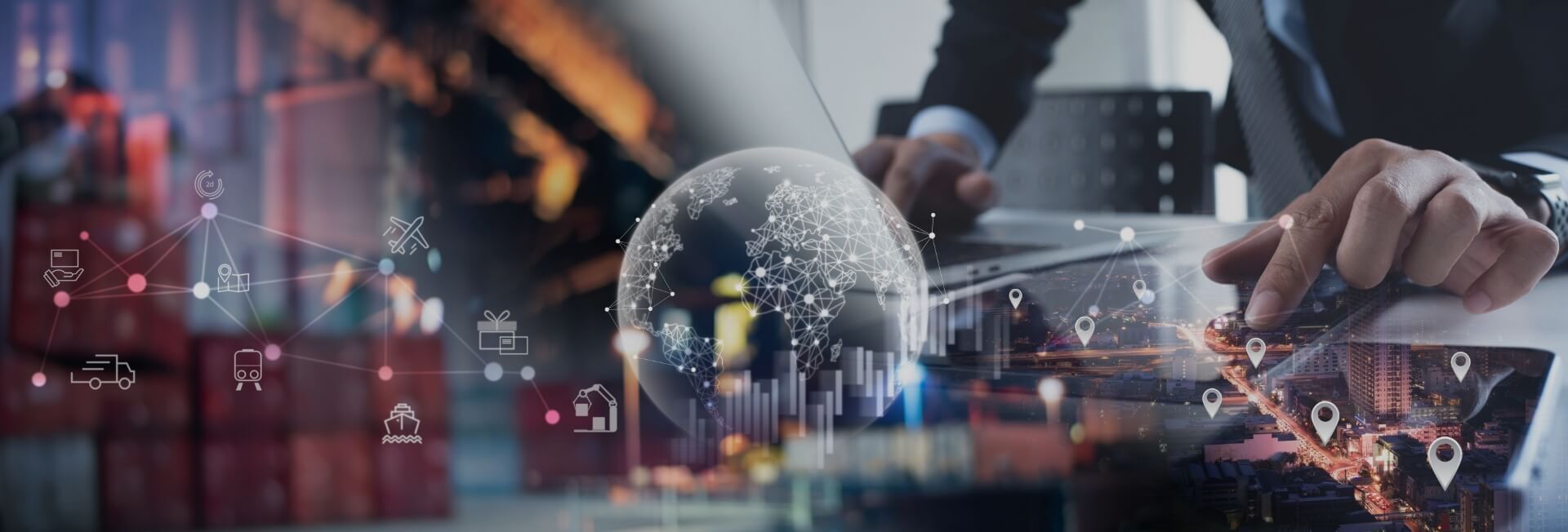
TMS and the use of predictive analytics for demand forecasting in logistics
- Home
- Resources
- Transportation Operations
- TMS and the use of predictive analytics for demand forecasting in logistics
Introduction
In today's fast-paced business environment, logistics management plays a crucial role in ensuring the smooth flow of goods and services. The ability to accurately forecast demand is essential for logistics companies to optimize their supply chains and meet customer expectations. Traditional methods of demand forecasting often fall short in providing accurate and timely insights. However, with the advent of Transportation Management Systems (TMS) and predictive analytics, logistics companies now have powerful tools at their disposal to improve their demand forecasting capabilities.
The Role of TMS in Logistics Management
Transportation Management Systems (TMS) are software solutions that help logistics companies streamline their operations and optimize their supply chains. TMS software provides functionalities such as load planning, carrier selection, route optimization, and freight auditing. By automating these processes, TMS solutions enable logistics companies to reduce costs, improve efficiency, and enhance customer service.
TMS software also facilitates real-time visibility into the supply chain, allowing logistics managers to track shipments, monitor carrier performance, and identify bottlenecks. This visibility is crucial for effective demand forecasting, as it provides valuable insights into the movement of goods and the performance of carriers. By integrating TMS with other supply chain management systems, such as Warehouse Management Systems (WMS) and Enterprise Resource Planning (ERP) systems, logistics companies can further enhance their demand forecasting capabilities.
Predictive Analytics: Enhancing Demand Forecasting
Predictive analytics is a branch of data analytics that uses historical data, statistical algorithms, and machine learning techniques to make predictions about future events. By analyzing patterns and trends in data, predictive analytics can provide valuable insights into customer behavior, market trends, and demand patterns. In the context of logistics, predictive analytics can be used to forecast demand for products, optimize inventory levels, and plan transportation routes.
By integrating predictive analytics capabilities into TMS software, logistics companies can leverage their vast amounts of data to improve their demand forecasting accuracy. TMS solutions can analyze historical shipment data, customer orders, market trends, and other relevant data sources to identify patterns and make predictions about future demand. This enables logistics companies to proactively adjust their supply chain operations, allocate resources efficiently, and meet customer demands in a timely manner.
Benefits of Using TMS and Predictive Analytics for Demand Forecasting
The integration of TMS and predictive analytics offers several benefits for logistics companies in terms of demand forecasting:
1. Improved Accuracy
By leveraging predictive analytics, TMS solutions can provide more accurate demand forecasts compared to traditional methods. The algorithms and machine learning models used in predictive analytics can analyze large volumes of data and identify patterns that may not be apparent to human analysts. This results in more accurate predictions and better-informed decision-making.
2. Timely Insights
Predictive analytics can provide real-time insights into demand patterns, allowing logistics companies to react quickly and adjust their operations accordingly. By identifying potential demand fluctuations in advance, logistics managers can allocate resources, plan transportation routes, and optimize inventory levels in a timely manner. This helps to prevent stockouts, minimize excess inventory, and improve overall supply chain efficiency.
3. Cost Savings
Accurate demand forecasting enables logistics companies to optimize their supply chain operations and reduce costs. By aligning inventory levels with demand patterns, logistics managers can avoid excess inventory and reduce carrying costs. Additionally, optimized transportation routes and load planning based on demand forecasts can help reduce fuel costs and improve overall transportation efficiency.
4. Enhanced Customer Service
Timely and accurate demand forecasting enables logistics companies to meet customer expectations and provide superior service. By ensuring that the right products are available at the right time and in the right quantities, logistics companies can enhance customer satisfaction and loyalty. This can lead to increased repeat business and positive word-of-mouth referrals.
Conclusion
The combination of TMS and predictive analytics is revolutionizing demand forecasting in logistics. By leveraging the power of data analytics and machine learning, logistics companies can improve the accuracy of their demand forecasts, gain timely insights, achieve cost savings, and enhance customer service. As the industry continues to evolve, the integration of TMS and predictive analytics will become increasingly essential for logistics companies to stay competitive in the market.