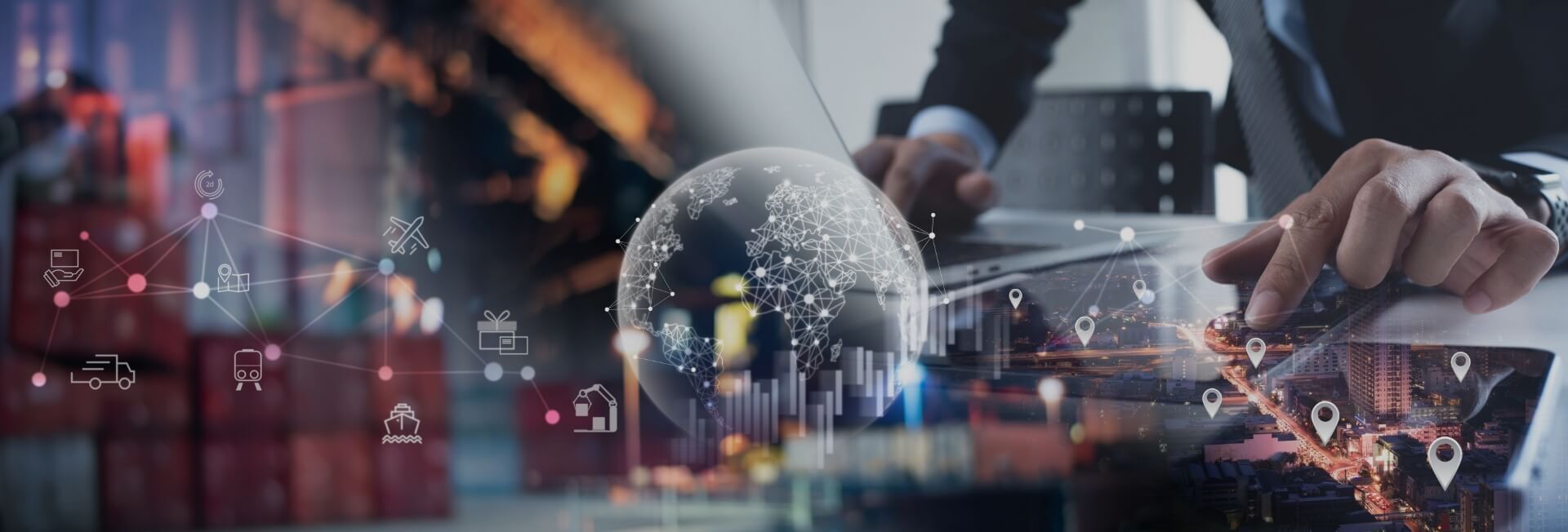
The role of predictive modeling in logistics optimization
- Home
- Resources
- Transportation Operations
- The role of predictive modeling in logistics optimization
The Power of Predictive Modeling in Logistics Optimization
Logistics management is a critical aspect of any business that involves the movement of goods. It involves planning, implementing, and controlling the efficient and effective flow of goods from the point of origin to the point of consumption. In today's fast-paced and competitive business environment, logistics optimization is essential to ensure the smooth operation of the supply chain and minimize costs.
The Need for Logistics Optimization
Supply chain optimization has become increasingly important as businesses strive to streamline their operations and achieve higher levels of efficiency. Logistics optimization involves finding the most cost-effective and time-efficient ways to move goods from one location to another. This includes optimizing transportation routes, minimizing transportation costs, and reducing delivery times.
Traditionally, logistics optimization relied on manual planning and decision-making processes. However, with the advent of technology and the availability of data, businesses now have access to advanced tools and techniques that can help them optimize their logistics operations. One such technique is predictive modeling.
What is Predictive Modeling?
Predictive modeling is a process that involves using historical data and statistical algorithms to make predictions about future events or outcomes. In the context of logistics optimization, predictive modeling can be used to forecast demand, predict transportation costs, and optimize routes and schedules.
By analyzing historical data and identifying patterns and trends, businesses can gain valuable insights into their logistics operations. These insights can then be used to make informed decisions and take proactive measures to optimize the supply chain.
The Benefits of Predictive Modeling in Logistics Optimization
There are several benefits of incorporating predictive modeling into logistics optimization:
1. Improved Planning and Decision-Making:
Predictive modeling provides businesses with valuable insights that can help them make more accurate and informed decisions. By predicting demand and optimizing routes and schedules, businesses can better plan their logistics operations and allocate resources more efficiently. This can lead to cost savings and improved customer satisfaction.
2. Enhanced Efficiency and Productivity:
By leveraging predictive modeling, businesses can identify inefficiencies in their logistics operations and take proactive measures to address them. For example, by analyzing transportation data, businesses can identify bottlenecks and optimize routes to ensure timely delivery. This can result in improved efficiency and productivity.
3. Cost Reduction:
Logistics optimization is all about finding the most cost-effective ways to move goods. Predictive modeling can help businesses identify cost-saving opportunities by analyzing transportation costs, optimizing routes, and minimizing fuel consumption. By reducing costs, businesses can improve their bottom line and gain a competitive advantage.
4. Improved Customer Service:
With predictive modeling, businesses can better predict demand and plan their logistics operations accordingly. This can result in faster delivery times and improved customer service. By meeting customer expectations and delivering goods on time, businesses can enhance customer satisfaction and loyalty.
Implementing Predictive Modeling in Logistics Optimization
Implementing predictive modeling in logistics optimization requires the following steps:
1. Data Collection and Analysis:
The first step in implementing predictive modeling is to collect and analyze relevant data. This includes historical transportation data, customer demand data, and other relevant information. By analyzing this data, businesses can identify patterns and trends that can be used to make predictions.
2. Model Development:
Once the data has been analyzed, businesses can develop predictive models using statistical algorithms and machine learning techniques. These models can then be used to make predictions about future events or outcomes.
3. Testing and Validation:
Before implementing predictive models in real-world logistics operations, it is important to test and validate the models. This involves comparing the predictions made by the models with the actual outcomes to assess their accuracy and reliability.
4. Integration and Implementation:
Once the predictive models have been tested and validated, they can be integrated into the logistics management system and implemented in real-world operations. This may involve integrating the predictive models with a transportation management system (TMS) software or other logistics automation tools.
5. Continuous Monitoring and Improvement:
Predictive modeling is an ongoing process that requires continuous monitoring and improvement. As new data becomes available and business conditions change, the predictive models need to be updated and refined to ensure their accuracy and effectiveness.
Conclusion
Predictive modeling is a powerful tool that can greatly enhance logistics optimization. By leveraging historical data and statistical algorithms, businesses can gain valuable insights into their logistics operations and make informed decisions to improve efficiency, reduce costs, and enhance customer service. As technology continues to advance, predictive modeling is likely to play an increasingly important role in logistics optimization, helping businesses stay competitive in today's fast-paced business environment.