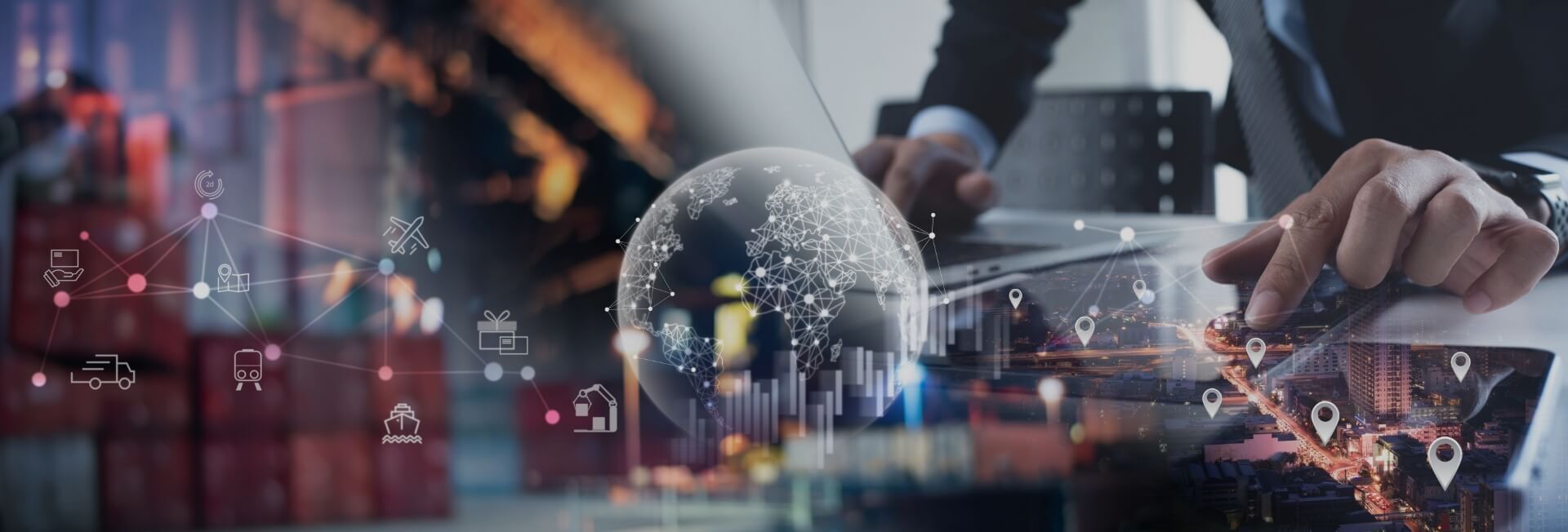
The role of predictive analytics in demand forecasting in a transportation management system
- Home
- Resources
- Transportation Operations
- The role of predictive analytics in demand forecasting in a transportation management system
The Power of Predictive Analytics in Demand Forecasting
In the world of logistics management, accurate demand forecasting is crucial for optimizing supply chain operations and ensuring timely delivery of goods. With the advent of technology, transportation management systems (TMS) have become a key tool in streamlining logistics processes. TMS software offers a range of functionalities that encompass supply chain optimization, logistics automation, and carrier relationship management. However, one of the most powerful features of a TMS is its ability to leverage predictive analytics for demand forecasting.
What is Predictive Analytics?
Predictive analytics is a branch of advanced analytics that uses historical data, statistical algorithms, and machine learning techniques to predict future outcomes. In the context of a transportation management system, predictive analytics can analyze historical shipment data, market trends, and other relevant factors to forecast demand accurately. This enables logistics managers to make informed decisions regarding inventory management, transportation capacity, and resource allocation.
The Benefits of Predictive Analytics in Demand Forecasting
Integrating predictive analytics into a TMS offers several benefits for demand forecasting and overall supply chain management:
1. Improved Accuracy
Predictive analytics algorithms can analyze large volumes of data and identify patterns that human analysts may miss. By considering various factors such as historical sales data, customer behavior, and market trends, predictive analytics models can provide more accurate demand forecasts compared to traditional methods. This helps in avoiding stockouts or overstocking, optimizing inventory levels, and reducing carrying costs.
2. Enhanced Efficiency
With accurate demand forecasts, logistics managers can optimize transportation planning and resource allocation. By knowing the expected demand, they can plan the most efficient routes, consolidate shipments, and make better use of available transportation capacity. This leads to reduced transportation costs, improved delivery times, and increased customer satisfaction.
3. Real-Time Visibility
Predictive analytics in a TMS provides real-time visibility into demand patterns and market dynamics. This allows logistics managers to quickly respond to changes in demand, adapt their supply chain strategies, and make informed decisions to mitigate risks. Real-time visibility enables proactive supply chain management, reducing the impact of disruptions and ensuring smooth operations.
4. Optimal Inventory Management
Accurate demand forecasting is essential for effective inventory management. By leveraging predictive analytics, logistics managers can determine the optimal inventory levels based on anticipated demand. This helps in minimizing inventory holding costs while ensuring sufficient stock availability to meet customer demands. Additionally, predictive analytics can identify slow-moving or obsolete inventory, enabling timely actions to prevent losses.
Challenges and Considerations
While predictive analytics offers significant benefits for demand forecasting in a TMS, there are some challenges and considerations to keep in mind:
1. Data Quality and Integration
The accuracy and reliability of predictive analytics models heavily depend on the quality of data used for analysis. It is essential to ensure that the TMS is integrated with reliable data sources and that the data is clean, consistent, and up-to-date. Inaccurate or incomplete data can lead to inaccurate forecasts and unreliable decision-making.
2. Continuous Model Improvement
Predictive analytics models need to be continuously updated and improved to account for changing market dynamics and new data. Regular monitoring and evaluation of the forecasting models are necessary to ensure their effectiveness. This may require collaboration between data analysts, logistics managers, and IT teams to refine the models and incorporate new variables as needed.
3. Human Expertise
While predictive analytics can provide valuable insights, human expertise is still essential in interpreting and contextualizing the results. Logistics managers should work closely with data analysts to understand the underlying assumptions and limitations of the forecasting models. This collaboration ensures that the forecasts are used effectively and align with the overall business goals and strategies.
Conclusion
The integration of predictive analytics in a transportation management system offers significant advantages for demand forecasting and overall supply chain optimization. By leveraging historical data, statistical algorithms, and machine learning techniques, logistics managers can accurately predict future demand, improve efficiency, optimize inventory management, and respond proactively to market dynamics. However, it is crucial to address data quality, continuously refine the forecasting models, and combine human expertise with analytics insights for effective decision-making. With the power of predictive analytics, logistics managers can stay ahead of the competition and achieve streamlined operations in today's dynamic and fast-paced business environment.