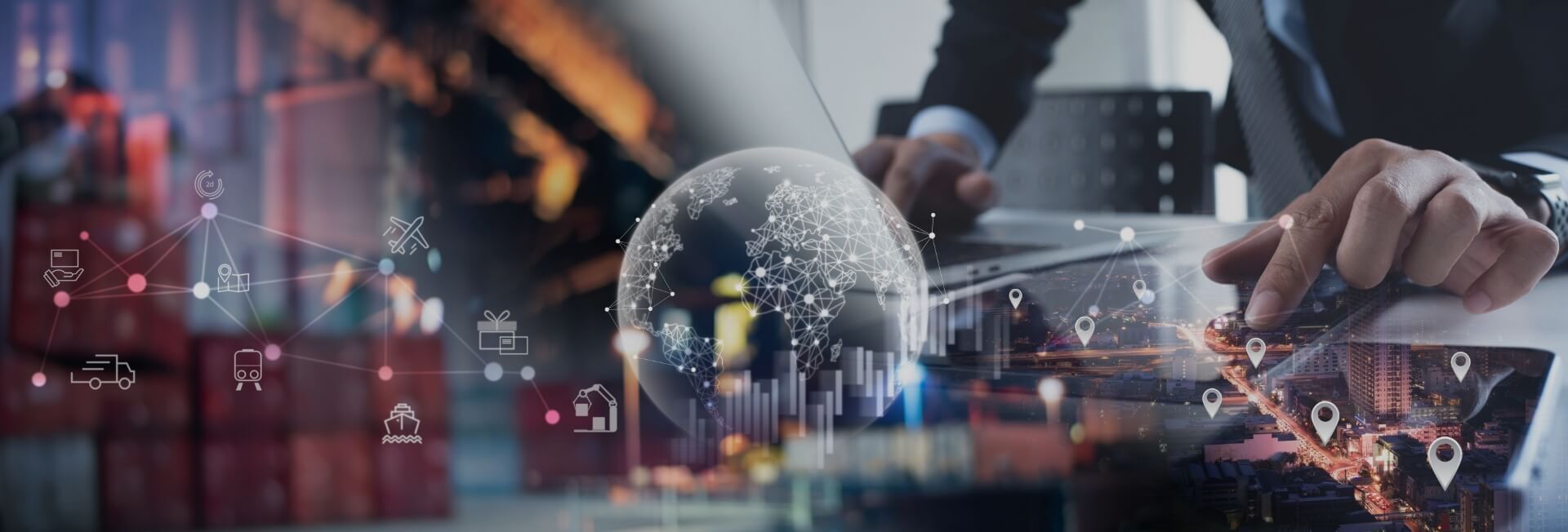
The role of machine learning in carrier TMS for predictive capacity planning
- Home
- Resources
- Transportation Operations
- The role of machine learning in carrier TMS for predictive capacity planning
The Power of Machine Learning in Carrier TMS for Predictive Capacity Planning
The logistics management industry has experienced significant advancements in recent years, with technology playing a crucial role in streamlining operations and optimizing supply chain processes. One of the key technologies that has emerged as a game-changer is machine learning. Machine learning, a subset of artificial intelligence, enables computer systems to learn and improve from experience without being explicitly programmed. In the context of carrier transportation management systems (TMS), machine learning algorithms can analyze vast amounts of data to predict capacity requirements, optimize route planning, and enhance carrier relationship management.
What is a Carrier TMS?
A carrier TMS, or transportation management system, is a software solution that helps businesses manage their transportation operations. It provides a centralized platform for planning, executing, and optimizing the movement of goods from one location to another. The system automates various tasks, such as order management, route planning, carrier selection, load optimization, and freight payment. A carrier TMS offers real-time visibility into the supply chain, allowing businesses to make data-driven decisions and improve overall logistics efficiency.
The Need for Predictive Capacity Planning
Effective capacity planning is crucial for businesses to meet customer demand while minimizing costs and maintaining high service levels. Traditionally, capacity planning in transportation relied on historical data and manual analysis. However, this approach often led to inefficiencies, as it failed to account for dynamic market conditions and changing customer demands. By leveraging machine learning algorithms, carrier TMS solutions can now perform predictive capacity planning, enabling businesses to anticipate capacity requirements and optimize resource allocation.
How Machine Learning Enables Predictive Capacity Planning
Machine learning algorithms analyze historical and real-time data to identify patterns and trends, enabling businesses to predict future capacity needs accurately. These algorithms consider various factors, such as historical shipment volumes, seasonality, market trends, and customer demand patterns. By integrating machine learning capabilities into carrier TMS software, businesses can optimize their transportation operations, reduce costs, and improve customer satisfaction.
Optimizing Route Planning and Load Optimization
Another significant benefit of machine learning in carrier TMS is optimizing route planning and load optimization. Machine learning algorithms can analyze vast amounts of data, such as traffic patterns, road conditions, and delivery locations, to determine the most efficient routes for transporting goods. By considering various parameters, such as delivery time windows, carrier capabilities, and service level agreements, the algorithms can optimize load allocation and minimize empty miles.
Furthermore, machine learning algorithms can adapt and learn from real-time data, enabling the system to make dynamic route adjustments based on changing conditions, such as traffic congestion or weather disruptions. This flexibility and adaptability help businesses improve transportation efficiency, reduce fuel consumption, and enhance overall supply chain performance.
Enhancing Carrier Relationship Management
Building and maintaining strong relationships with carriers is essential for businesses to ensure reliable and efficient transportation services. Machine learning algorithms can play a significant role in enhancing carrier relationship management by analyzing carrier performance data and identifying opportunities for improvement.
By tracking carrier performance metrics, such as on-time delivery, transit times, and service quality, machine learning algorithms can identify carriers that consistently meet or exceed performance expectations. This information can help businesses make data-driven decisions when selecting carriers for specific shipments, leading to improved service levels and reduced risk of disruptions.
Machine learning algorithms can also detect patterns of carrier performance issues, such as frequent delays or damages, and proactively address these issues through targeted interventions. By identifying areas for improvement and working collaboratively with carriers, businesses can enhance overall supply chain performance and foster long-term partnerships.
Integrating TMS Software with Machine Learning Capabilities
To leverage the power of machine learning in carrier TMS, businesses need to integrate TMS software with machine learning capabilities seamlessly. This integration allows the TMS to collect and analyze vast amounts of data from various sources, such as historical shipment data, real-time tracking information, weather forecasts, and market trends.
Furthermore, it is essential to ensure the accuracy and quality of the data used by machine learning algorithms. Data cleansing and normalization processes should be in place to eliminate outliers and ensure consistent data inputs. By maintaining clean and accurate data, businesses can improve the reliability and effectiveness of the machine learning algorithms.
The Future of Carrier TMS with Machine Learning
The role of machine learning in carrier TMS is expected to grow rapidly in the coming years. As technology continues to advance, machine learning algorithms will become more sophisticated and capable of handling complex supply chain challenges. The integration of machine learning with other emerging technologies, such as Internet of Things (IoT) devices and blockchain, will further enhance the capabilities of carrier TMS solutions.
With predictive capacity planning, optimized route planning, and enhanced carrier relationship management, businesses can achieve significant cost savings, improved operational efficiency, and better customer satisfaction. By embracing machine learning in carrier TMS, businesses can stay ahead of the competition and navigate the complexities of the modern logistics landscape.
Conclusion
Machine learning is revolutionizing the carrier TMS industry by enabling predictive capacity planning, optimizing route planning, and enhancing carrier relationship management. By leveraging machine learning algorithms, businesses can make data-driven decisions, improve operational efficiency, and enhance customer satisfaction. Integrating machine learning capabilities into carrier TMS software is essential for businesses seeking to stay ahead in the rapidly evolving logistics landscape. As technology continues to advance, the role of machine learning in carrier TMS is only expected to grow, bringing further advancements and opportunities for the supply chain industry.