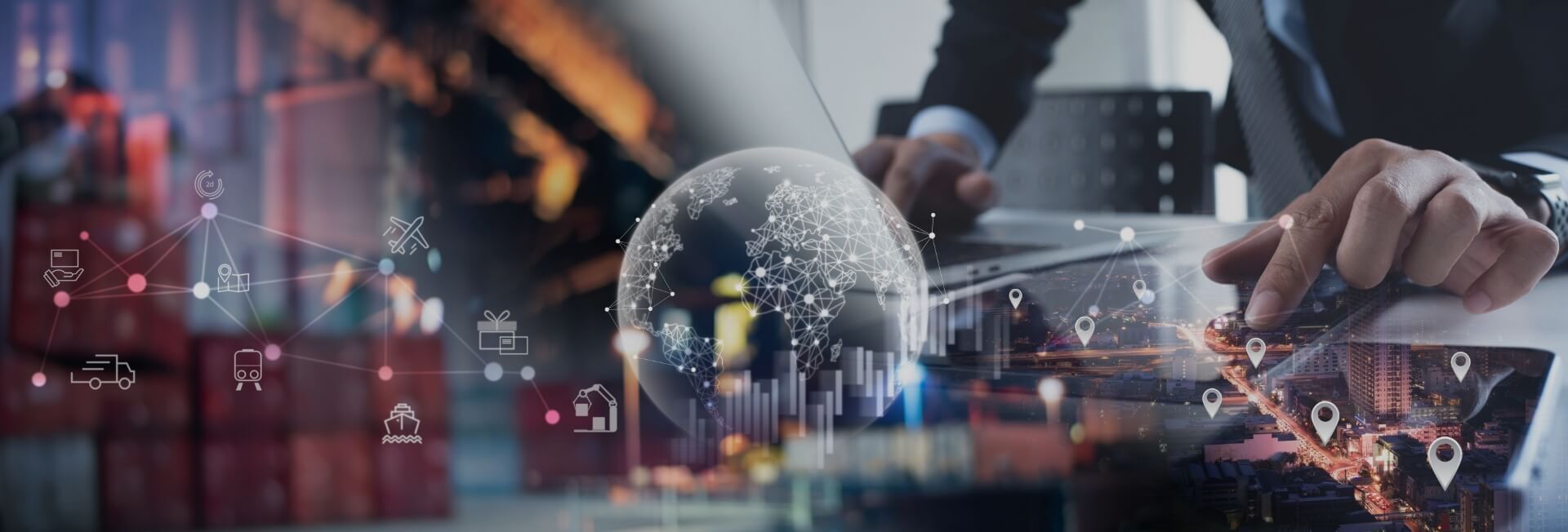
The role of data analytics in demand forecasting for logistics
- Home
- Resources
- Transportation Operations
- The role of data analytics in demand forecasting for logistics
The Power of Data Analytics in Demand Forecasting for Logistics
Logistics management is a critical component of any supply chain, ensuring the smooth flow of goods from point of origin to final destination. Effective logistics management requires accurate demand forecasting, as it helps to optimize transportation, reduce costs, and improve overall customer satisfaction. In today's digital age, data analytics plays a crucial role in demand forecasting for logistics, enabling businesses to make more informed decisions and stay ahead of the competition.
The Importance of Demand Forecasting in Logistics
Demand forecasting is the process of estimating the future demand for a product or service. In the context of logistics, demand forecasting helps businesses determine how much inventory to stock, how many transportation resources to allocate, and how to plan for future demand fluctuations.
Accurate demand forecasting is essential for optimizing logistics operations. By forecasting demand accurately, businesses can avoid stockouts and overstocking, reduce transportation costs, and improve customer satisfaction. It also helps businesses plan their resources more efficiently, ensuring that they have the right amount of inventory and transportation capacity to meet customer demand.
Data Analytics: Transforming Demand Forecasting in Logistics
Data analytics has revolutionized the field of demand forecasting in logistics. With the advent of advanced analytics techniques and the availability of large volumes of data, businesses can now leverage data to gain valuable insights and make more accurate demand forecasts.
There are several ways in which data analytics is transforming demand forecasting in logistics:
1. Improved Accuracy
Data analytics allows businesses to analyze historical sales data, market trends, and other relevant factors to make more accurate demand forecasts. By identifying patterns and correlations in the data, businesses can better understand customer behavior and predict future demand with greater precision.
For example, using advanced machine learning algorithms, businesses can analyze historical sales data along with external factors such as weather patterns, holidays, and economic indicators to forecast demand for seasonal products. This allows businesses to optimize their inventory and transportation resources, ensuring that they have the right amount of stock and capacity to meet customer demand during peak seasons.
2. Real-Time Demand Forecasting
Traditionally, demand forecasting was done on a monthly or quarterly basis, using historical sales data and manual calculations. However, with data analytics, businesses can now perform real-time demand forecasting, allowing them to respond quickly to changes in customer demand.
Real-time demand forecasting enables businesses to adjust their inventory levels and transportation resources dynamically, based on the latest market conditions. For example, if there is a sudden increase in demand for a particular product, businesses can quickly allocate more resources to meet the demand, ensuring that they can fulfill customer orders on time.
3. Supply Chain Optimization
Data analytics not only helps businesses forecast demand accurately but also enables them to optimize their supply chain. By analyzing data from various sources such as suppliers, warehouses, and transportation providers, businesses can identify bottlenecks and inefficiencies in their supply chain and take proactive measures to address them.
For example, using data analytics, businesses can identify suppliers with the shortest lead times, warehouses with the highest storage capacity, and transportation providers with the best on-time delivery performance. This information allows businesses to make more informed decisions when it comes to selecting suppliers, optimizing inventory levels, and choosing the most efficient transportation routes.
4. Enhanced Customer Satisfaction
Accurate demand forecasting is directly linked to customer satisfaction. By accurately predicting customer demand, businesses can ensure that they have the right products in stock and can fulfill customer orders promptly. This leads to improved customer satisfaction and loyalty.
Data analytics enables businesses to analyze customer data, such as purchase history, preferences, and feedback, to gain insights into customer behavior and preferences. By understanding customer needs and preferences better, businesses can tailor their offerings and services to meet customer expectations, leading to higher customer satisfaction.
Conclusion
Data analytics has revolutionized demand forecasting in logistics, enabling businesses to make more accurate predictions and optimize their operations. By leveraging data analytics, businesses can improve the accuracy of their demand forecasts, perform real-time demand forecasting, optimize their supply chain, and enhance customer satisfaction. In today's competitive business landscape, data analytics is no longer a luxury but a necessity for logistics management. Businesses that embrace data analytics will gain a competitive edge and stay ahead of the curve.