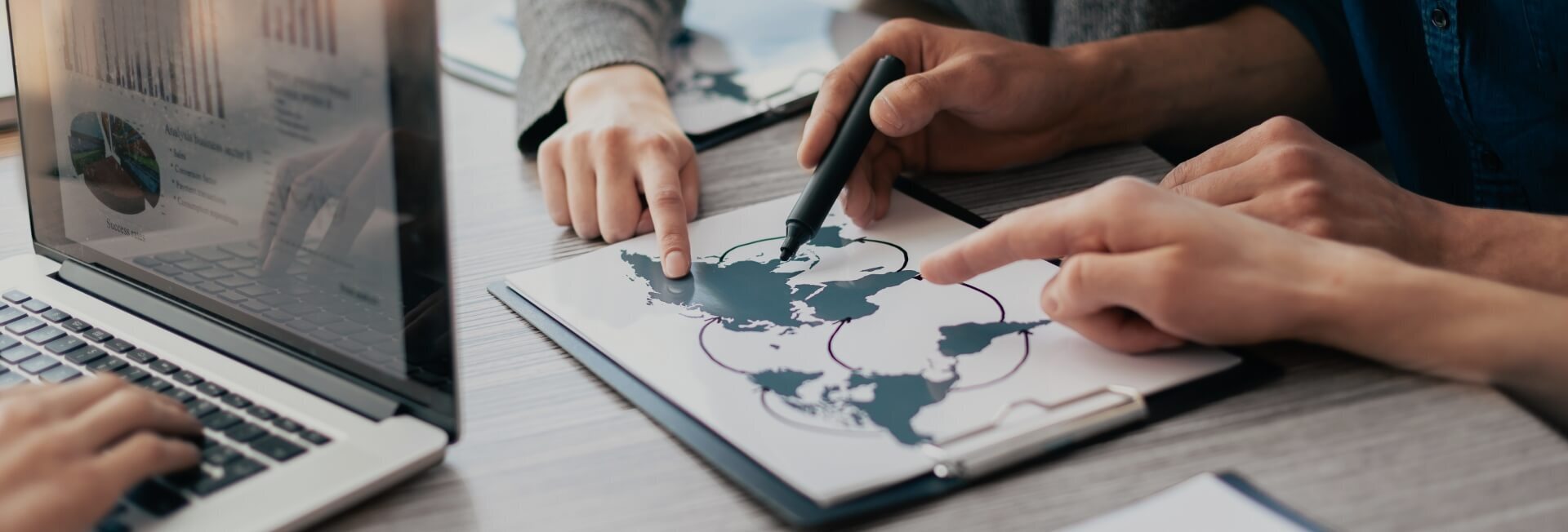
The role of machine learning in improving demand forecasting in freight logistics
- Home
- Resources
- Freight Logistics and Planning
- The role of machine learning in improving demand forecasting in freight logistics
The Role of Machine Learning in Revolutionizing Demand Forecasting in Freight Logistics
Freight logistics is a complex industry that involves the movement of goods from one location to another. Whether it's by land, sea, or air, the efficient management of freight is crucial for businesses to meet customer demand and maintain a competitive edge. However, accurately forecasting demand for freight can be a significant challenge due to various factors such as changing consumer preferences, market trends, and unpredictable events like weather disruptions or global crises.
Traditional methods of demand forecasting in freight logistics have relied on historical data analysis and statistical models. While these approaches have provided some insights, they often fall short in capturing the dynamic and ever-evolving nature of the industry. This is where machine learning comes in.
What is Machine Learning?
Machine learning is a branch of artificial intelligence that focuses on the development of algorithms and models that can learn from and make predictions or decisions based on data. Rather than relying on explicitly programmed instructions, machine learning algorithms can automatically analyze vast amounts of data, identify patterns, and make predictions or take actions accordingly.
In the context of demand forecasting in freight logistics, machine learning algorithms can analyze historical shipping data, market trends, weather patterns, and various other factors to predict future demand accurately. By leveraging the power of machine learning, businesses can gain valuable insights into their supply chains and make data-driven decisions to optimize their freight operations.
The Benefits of Machine Learning in Demand Forecasting
Machine learning offers several key benefits that make it an ideal tool for demand forecasting in freight logistics:
- Improved Accuracy: Machine learning algorithms can analyze large volumes of data and identify complex patterns that may not be apparent to human analysts. This leads to more accurate demand forecasts, reducing the risk of overstocking or running out of inventory.
- Real-time Insights: Traditional demand forecasting methods often rely on historical data, which may not capture the latest market trends or disruptions. Machine learning algorithms can analyze real-time data, enabling businesses to adapt their freight operations quickly in response to changing market conditions.
- Cost Savings: By accurately forecasting demand, businesses can optimize their inventory levels, reduce storage costs, and minimize the need for expedited shipping or emergency logistics. This leads to significant cost savings and improved overall profitability.
- Enhanced Customer Service: Accurate demand forecasting enables businesses to meet customer demand more effectively. By ensuring the availability of products when and where customers need them, businesses can enhance customer satisfaction and loyalty.
Applications of Machine Learning in Freight Logistics
Machine learning is revolutionizing demand forecasting in freight logistics across various areas of the supply chain. Let's explore some of the key applications:
1. Freight Rate Management
Freight rate management involves determining the optimal pricing strategy for shipping services. Machine learning algorithms can analyze historical shipping data, market conditions, and other relevant factors to predict the most competitive and profitable rates. By accurately forecasting demand and understanding market dynamics, businesses can optimize their pricing strategies to attract customers while maximizing their profitability.
2. Freight Network Optimization
Freight network optimization aims to determine the most efficient routes, modes of transportation, and shipping schedules to minimize costs and maximize service levels. Machine learning algorithms can analyze historical shipping data, traffic patterns, weather conditions, and other variables to identify the optimal network configuration. By optimizing their freight networks, businesses can reduce transit times, lower fuel consumption, and improve overall operational efficiency.
3. Inventory Management
Effective inventory management is crucial for meeting customer demand while minimizing holding costs. Machine learning algorithms can analyze historical sales data, market trends, and other factors to predict future demand accurately. By integrating demand forecasts with inventory management systems, businesses can optimize their inventory levels, reduce stockouts, and improve order fulfillment rates.
4. Route Planning and Optimization
Efficient route planning is essential for minimizing transportation costs and ensuring timely deliveries. Machine learning algorithms can analyze historical traffic data, weather conditions, and other variables to identify the most optimal routes. Additionally, real-time data integration enables businesses to dynamically adjust routes in response to changing conditions, such as traffic congestion or road closures.
Challenges in Implementing Machine Learning for Demand Forecasting
While machine learning holds immense potential for revolutionizing demand forecasting in freight logistics, there are several challenges that businesses must overcome for successful implementation:
- Data Quality: Machine learning algorithms require high-quality, accurate, and relevant data for training and making accurate predictions. However, the data collected in the freight logistics industry can be incomplete, inconsistent, or of poor quality. Businesses need to invest in data cleansing and validation processes to ensure the reliability of their machine learning models.
- Data Integration: Freight logistics involves multiple stakeholders, each generating and managing their own data. Integrating data from various sources, such as carriers, suppliers, and customers, can be a significant challenge. Businesses need to establish robust data integration processes and infrastructure to ensure seamless data flow across the supply chain.
- Change Management: Implementing machine learning for demand forecasting requires a cultural shift within the organization. Employees need to embrace data-driven decision-making and be open to leveraging machine learning technologies. Change management strategies, such as training programs and communication initiatives, are crucial for successful implementation.
- Algorithm Selection: There are various machine learning algorithms available, each with its strengths and limitations. Selecting the most appropriate algorithm for a specific demand forecasting task requires a deep understanding of the problem domain and the capabilities of different algorithms. Businesses need to invest in skilled data science resources or partner with experienced machine learning providers to ensure optimal algorithm selection.
The Future of Demand Forecasting in Freight Logistics
As technology continues to advance, the role of machine learning in demand forecasting in freight logistics will only become more prominent. With advancements in areas such as big data analytics, internet of things (IoT), and cloud computing, machine learning algorithms will have access to even larger volumes of data, enabling more accurate and timely predictions.
Additionally, the integration of machine learning with other cutting-edge technologies such as blockchain and robotic process automation (RPA) will further enhance the efficiency and transparency of freight logistics operations. Blockchain can provide secure and immutable records of transactions, while RPA can automate repetitive tasks, freeing up human resources for more strategic and value-added activities.
In conclusion, machine learning is revolutionizing demand forecasting in freight logistics, enabling businesses to make data-driven decisions and optimize their shipping operations. By accurately predicting demand, businesses can reduce costs, improve customer service, and gain a competitive edge in the fast-paced world of modern freight management. Embracing machine learning and other innovative technologies is crucial for businesses looking to thrive in the ever-evolving freight logistics landscape.
Related Blogs
The impact of real-time temperature monitoring on ensuring product quality in freight transportation
Read More