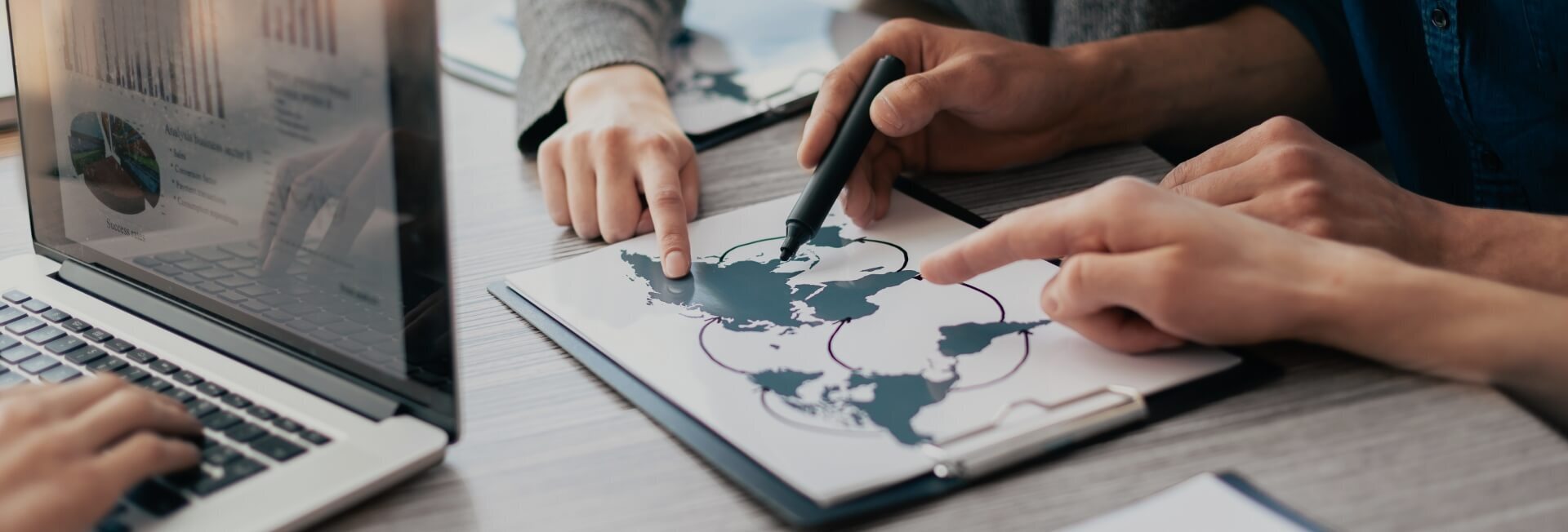
The impact of machine learning algorithms on optimizing freight consolidation and load planning in logistics
- Home
- Resources
- Freight Logistics and Planning
- The impact of machine learning algorithms on optimizing freight consolidation and load planning in logistics
Introduction
The logistics industry is constantly evolving, driven by technological advancements and the need for more efficient and cost-effective solutions. One area that has seen significant innovation is freight consolidation and load planning. Traditionally, this process involved manual calculations and guesswork, leading to suboptimal results and wasted resources. However, with the advent of machine learning algorithms, the industry is experiencing a revolution in optimizing freight consolidation and load planning.
The Role of Machine Learning Algorithms
Machine learning algorithms are a subset of artificial intelligence that allow computers to learn from data and make predictions or take actions without being explicitly programmed. In the context of freight consolidation and load planning, these algorithms analyze historical data, real-time information, and various other factors to optimize the allocation of resources and improve overall efficiency.
Benefits of Machine Learning in Freight Consolidation
Implementing machine learning algorithms in freight consolidation and load planning offers several benefits for logistics companies:
1. Improved Efficiency and Cost Savings
Machine learning algorithms have the ability to analyze large amounts of data and make complex calculations in a fraction of the time it would take a human. This leads to faster and more accurate decision-making, resulting in improved efficiency and cost savings. By optimizing the allocation of resources, companies can reduce the number of trucks needed, minimize empty miles, and decrease fuel consumption.
2. Enhanced Freight Tracking and Visibility
With the integration of machine learning algorithms, freight tracking becomes more precise and reliable. Real-time data on the location, condition, and status of shipments can be collected and analyzed to provide accurate updates to customers and stakeholders. This level of visibility allows for better planning and coordination, reducing delays and improving customer satisfaction.
3. Intelligent Load Planning
Load planning is a critical aspect of freight consolidation, ensuring that trucks are filled to capacity and resources are utilized efficiently. Machine learning algorithms can analyze historical data on shipment sizes, weight distribution, and delivery patterns to generate optimized load plans. By considering various constraints such as delivery deadlines and vehicle capacities, these algorithms can create intelligent load plans that minimize wasted space and maximize utilization.
4. Predictive Analytics for Demand Forecasting
Machine learning algorithms can also be used for demand forecasting, helping logistics companies anticipate future shipment volumes and plan their operations accordingly. By analyzing historical data and considering external factors such as seasonality and market trends, these algorithms can provide accurate predictions and enable proactive decision-making. This allows companies to optimize their resources and adapt to changing market conditions, reducing costs and improving customer service.
Challenges and Considerations
While machine learning algorithms offer significant benefits, there are also challenges and considerations to keep in mind:
1. Data Quality and Availability
Machine learning algorithms rely heavily on data, and the quality and availability of data can greatly impact their performance. It is crucial for logistics companies to have access to accurate and relevant data for training and testing these algorithms. This may require investments in data collection and integration systems.
2. Integration with Existing Systems
Implementing machine learning algorithms for freight consolidation and load planning may require integration with existing systems such as transportation management software. This can be a complex process that requires careful planning and coordination to ensure a seamless transition and maximum effectiveness.
3. Human Expertise and Oversight
While machine learning algorithms can automate many aspects of freight consolidation and load planning, human expertise and oversight are still essential. Logistics professionals play a critical role in setting constraints, defining objectives, and validating the output of these algorithms. They bring domain knowledge and intuition that algorithms alone may not possess.
The Future of Freight Consolidation and Load Planning
The impact of machine learning algorithms on optimizing freight consolidation and load planning is just the beginning. As technology continues to advance, we can expect further innovations in this field:
1. Integration with IoT and Sensor Technology
The Internet of Things (IoT) and sensor technology are revolutionizing the logistics industry. By integrating machine learning algorithms with IoT devices and sensors, companies can gather real-time data on factors such as temperature, humidity, and vibration. This data can be used to optimize load planning and ensure the integrity of sensitive shipments.
2. Collaboration and Network Optimization
Machine learning algorithms have the potential to enable collaboration and network optimization among multiple logistics providers. By sharing data and insights, companies can optimize routes, consolidate shipments, and reduce costs. This collaborative approach can lead to more efficient and sustainable supply chains.
3. Automation and Autonomous Vehicles
The rise of automation and autonomous vehicles is transforming the logistics landscape. Machine learning algorithms can play a crucial role in optimizing the movement of autonomous vehicles and coordinating deliveries. This can lead to increased efficiency, reduced congestion, and improved safety on the roads.
Conclusion
Machine learning algorithms are revolutionizing the logistics industry by optimizing freight consolidation and load planning. These algorithms offer numerous benefits, including improved efficiency, enhanced freight tracking, intelligent load planning, and predictive analytics. However, challenges such as data quality and integration with existing systems need to be addressed. Looking ahead, the future of freight consolidation and load planning will likely involve integration with IoT and sensor technology, collaboration among logistics providers, and the rise of automation and autonomous vehicles. By embracing these advancements, logistics companies can stay ahead of the competition and deliver innovative freight solutions.