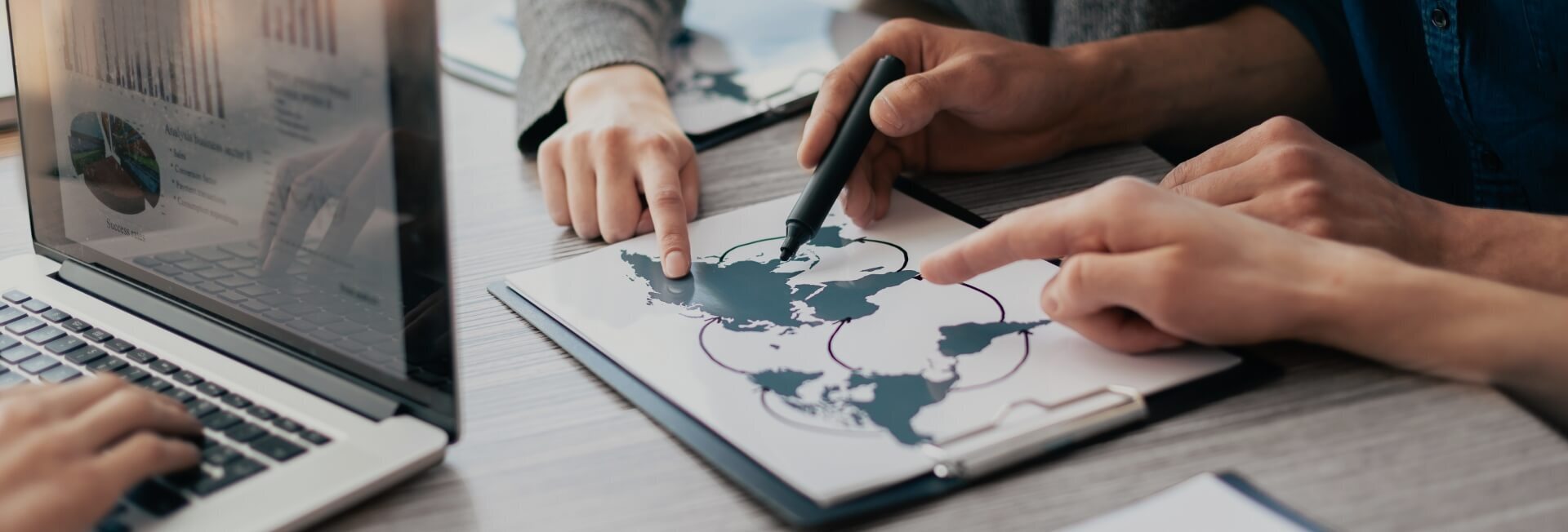
The challenges of managing data quality in freight tracking systems
- Home
- Resources
- Freight Logistics and Planning
- The challenges of managing data quality in freight tracking systems
The Challenges of Data Quality in Freight Tracking Systems
In today's rapidly evolving freight industry, the ability to track shipments in real-time has become a necessity. Freight tracking systems have revolutionized the way logistics companies operate, providing them with valuable insights into their supply chain and allowing them to optimize their operations for maximum efficiency. However, the success of these systems relies heavily on the quality of the data that is fed into them. Inaccurate or incomplete data can lead to costly errors and inefficiencies, making data quality a top priority for freight management companies.
The Importance of Data Quality in Freight Tracking
Accurate and reliable data is essential for effective freight tracking. By having real-time visibility into the location and status of shipments, logistics companies can make informed decisions about routing, scheduling, and resource allocation. This helps them optimize their operations and provide better service to their customers. In addition, accurate data allows companies to quickly identify and resolve any issues that may arise during the transportation process, minimizing delays and disruptions.
However, ensuring data quality in freight tracking systems can be challenging due to several factors:
1. Data Integration
Freight tracking systems rely on data from multiple sources, including carriers, shippers, and third-party logistics providers. Integrating data from these disparate sources can be complex, as each may have different data formats, standards, and protocols. Inconsistent or incompatible data can lead to errors and inaccuracies in the tracking system, compromising its effectiveness.
2. Data Accuracy
One of the biggest challenges in managing data quality in freight tracking systems is ensuring the accuracy of the data. The data collected may come from various sources, such as GPS devices, sensors, or manual inputs. Each of these sources has its own potential for error. For example, GPS devices can be affected by signal interference or inaccurate positioning, while manual inputs are prone to human error. Ensuring the accuracy of the data requires implementing robust validation and verification processes.
3. Data Completeness
Another challenge is ensuring data completeness. Freight tracking systems require a wide range of data points to provide comprehensive visibility into the supply chain. This includes information on the location, temperature, humidity, and condition of the goods being transported. If any of these data points are missing or incomplete, it can lead to gaps in the tracking information and hinder decision-making.
4. Data Timeliness
Timeliness is critical in freight tracking. Delayed or outdated data can result in missed opportunities or ineffective decision-making. For example, if a shipment is delayed, logistics companies need to be notified as soon as possible so they can take appropriate action. Ensuring the timely availability of data requires efficient data capture, transmission, and processing.
Addressing Data Quality Challenges
To overcome the challenges of managing data quality in freight tracking systems, logistics companies can implement the following strategies:
1. Standardize Data Formats and Protocols
Standardizing data formats and protocols across all stakeholders can greatly simplify data integration. This ensures that data from different sources can be seamlessly integrated into the tracking system, reducing the risk of errors and inconsistencies.
2. Implement Data Validation and Verification Processes
Validating and verifying the accuracy of the data is crucial. This can be done through automated processes that compare the tracking data against predefined rules and thresholds. Any discrepancies or anomalies can be flagged for further investigation and resolution.
3. Enhance Data Capture and Transmission
Improving the methods used to capture and transmit data can help ensure its timeliness. This can involve using advanced technologies such as IoT devices, RFID tags, or mobile apps to collect data in real-time and transmit it instantly to the tracking system. Automating data capture reduces the risk of manual errors and delays.
4. Implement Data Governance Frameworks
Data governance frameworks provide a structured approach to managing data quality. They define the roles, responsibilities, and processes for ensuring data accuracy, completeness, and timeliness. By implementing such frameworks, logistics companies can establish a culture of data quality and accountability.
Managing data quality in freight tracking systems is an ongoing process. As technology continues to advance and new data sources become available, logistics companies need to adapt and evolve their data management strategies. By investing in innovative freight solutions and advanced freight systems, they can stay ahead of the competition and deliver superior service to their customers.
Conclusion
The challenges of managing data quality in freight tracking systems are significant, but they can be overcome with the right strategies and technologies. By addressing issues related to data integration, accuracy, completeness, and timeliness, logistics companies can ensure that their tracking systems provide reliable and actionable insights. This, in turn, allows them to optimize their operations, improve customer satisfaction, and drive business growth in the modern freight management industry.
Related Blogs
The impact of real-time temperature monitoring on ensuring product quality in freight transportation
Read More