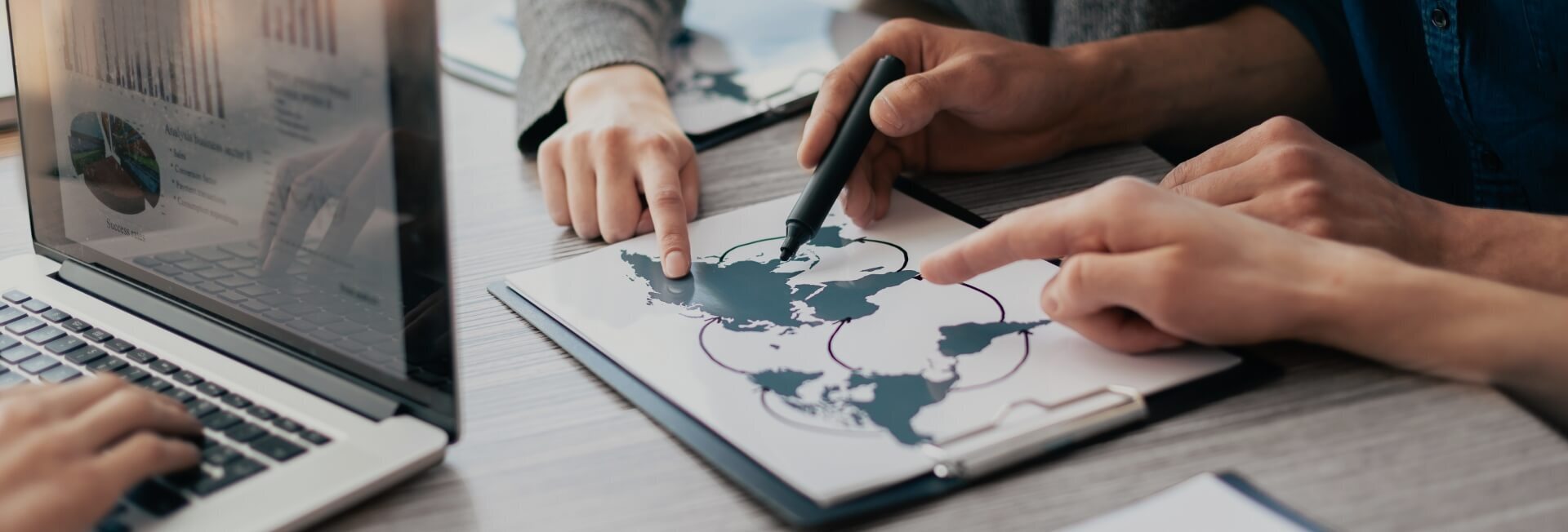
The benefits of using machine learning in demand forecasting
- Home
- Resources
- Freight Logistics and Planning
- The benefits of using machine learning in demand forecasting
The Power of Machine Learning in Demand Forecasting
Introduction
Freight logistics is an industry that heavily relies on accurate demand forecasting to optimize operations and ensure efficient transportation of goods. Traditional methods of demand forecasting can be time-consuming, prone to errors, and lack the ability to adapt to changing market conditions. However, with advancements in technology, machine learning has emerged as a powerful tool that can revolutionize the way demand forecasting is conducted in the freight logistics industry.
The Role of Machine Learning in Demand Forecasting
Machine learning is a subset of artificial intelligence that focuses on training algorithms to learn patterns and make predictions or decisions without being explicitly programmed. In the context of demand forecasting, machine learning algorithms analyze historical data and identify patterns and trends that can help predict future demand with a high degree of accuracy.
Benefits of Using Machine Learning in Demand Forecasting
Using machine learning in demand forecasting offers several benefits for freight logistics companies. Let's explore some of the key advantages:
1. Improved Forecast Accuracy
One of the main advantages of using machine learning in demand forecasting is the ability to achieve a higher level of accuracy compared to traditional methods. Machine learning algorithms can analyze large volumes of data, including historical sales data, market trends, weather patterns, and customer behavior, to identify patterns and make more accurate predictions. This can help freight logistics companies optimize their inventory levels, reduce stockouts, and improve customer satisfaction.
2. Real-Time Demand Forecasting
Traditional demand forecasting methods often rely on historical data and may not take into account real-time market conditions. Machine learning algorithms, on the other hand, can continuously analyze real-time data from various sources, such as social media, online reviews, and competitor pricing, to make more accurate and up-to-date demand forecasts. This enables freight logistics companies to respond quickly to changing market conditions and make informed decisions.
3. Demand Variability Analysis
Machine learning algorithms can also help freight logistics companies analyze demand variability and identify factors that contribute to fluctuations in demand. By understanding the underlying causes of demand variability, companies can develop strategies to mitigate its impact and optimize their operations. For example, if a certain product is highly affected by seasonal demand, the company can adjust its inventory levels and transportation capacity accordingly to avoid excess costs or stockouts.
4. Enhanced Supply Chain Efficiency
Machine learning algorithms can optimize supply chain operations by analyzing data from various sources, including transportation routes, warehouse capacities, and production schedules. By considering all these factors, machine learning algorithms can help freight logistics companies make more accurate demand forecasts, optimize inventory levels, and improve overall supply chain efficiency. This can result in cost savings, reduced lead times, and improved customer service.
Conclusion
Machine learning has the potential to revolutionize demand forecasting in the freight logistics industry. By leveraging the power of advanced algorithms and analyzing large volumes of data, freight logistics companies can achieve a higher level of accuracy in their demand forecasts, respond quickly to market changes, and optimize their operations. As the industry continues to embrace technology-driven solutions, machine learning will play a crucial role in shaping the future of freight logistics and delivering innovative solutions to meet the evolving needs of customers.
Related Blogs
The impact of real-time temperature monitoring on ensuring product quality in freight transportation
Read More