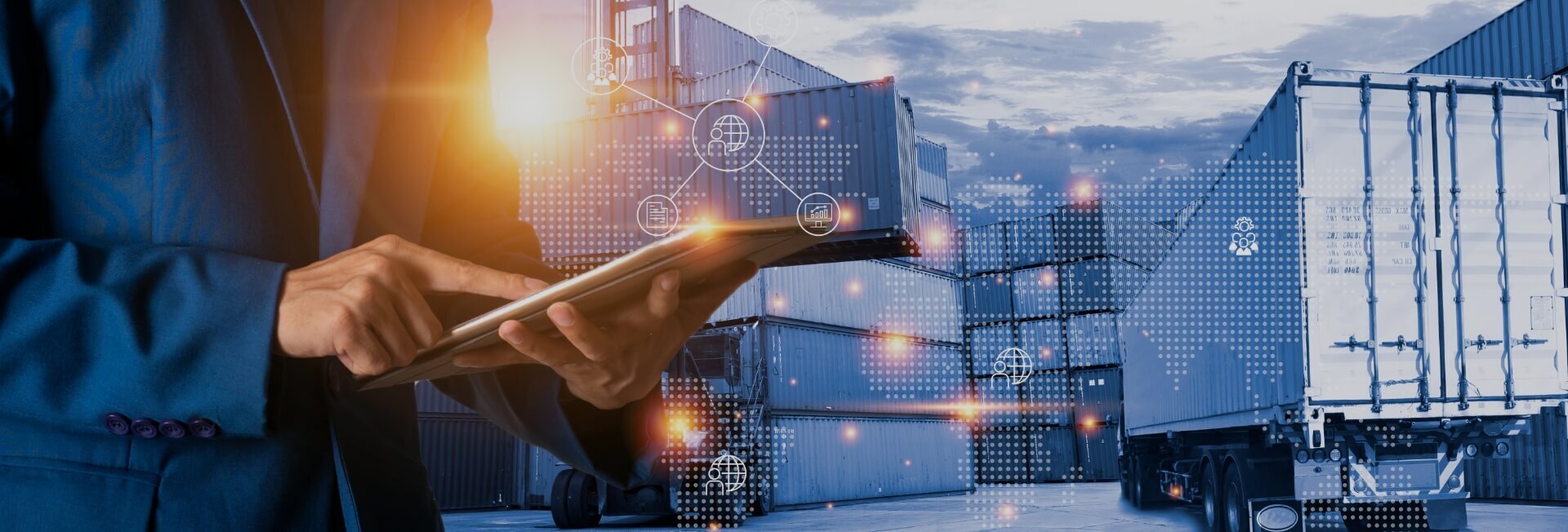
The role of predictive analytics in optimizing inventory levels for logistics automation
- Home
- Resources
- Fleet Operations and Optimization
- The role of predictive analytics in optimizing inventory levels for logistics automation
The Role of Predictive Analytics in Optimizing Inventory Levels for Logistics Automation
Logistics automation has revolutionized the supply chain industry, enabling businesses to streamline their operations and improve efficiency. One key aspect of logistics automation is the optimization of inventory levels, which involves accurately forecasting demand and ensuring the right amount of stock is available at the right time. This is where predictive analytics plays a crucial role.
What is Predictive Analytics?
Predictive analytics is the use of historical data, statistical algorithms, and machine learning techniques to make predictions about future events or behaviors. In the context of inventory optimization, predictive analytics uses historical sales data, customer demand patterns, market trends, and other relevant factors to forecast future demand accurately. By analyzing this data, businesses can make informed decisions about inventory levels, reducing the risk of stockouts or excess inventory.
The Benefits of Predictive Analytics in Inventory Optimization
Optimizing inventory levels through predictive analytics offers several benefits for businesses:
1. Improved Efficiency and Reduced Costs
By accurately predicting future demand, businesses can avoid stockouts and overstocking, which can lead to lost sales and increased carrying costs. With the right inventory levels, businesses can minimize inventory holding costs while ensuring products are available when customers need them. This leads to improved efficiency and reduced costs.
2. Enhanced Customer Satisfaction
With predictive analytics, businesses can better understand customer demand patterns and align their inventory levels accordingly. This ensures that customers can find the products they need when they need them, leading to higher customer satisfaction. Satisfied customers are more likely to become repeat customers, contributing to increased sales and business growth.
3. Optimal Allocation of Resources
Predictive analytics helps businesses allocate their resources effectively. By accurately forecasting demand, businesses can optimize their production schedules, allocate warehouse space efficiently, and plan transportation logistics. This eliminates unnecessary costs and ensures resources are utilized in the most efficient way possible.
4. Proactive Decision-Making
Predictive analytics enables businesses to make proactive decisions rather than reactive ones. By having a clear understanding of future demand, businesses can plan their procurement, production, and distribution activities in advance. This allows them to respond proactively to market changes, reduce lead times, and better serve their customers.
Implementing Predictive Analytics for Inventory Optimization
Implementing predictive analytics for inventory optimization involves the following steps:
1. Data Collection and Integration
The first step is to collect and integrate relevant data from various sources. This includes historical sales data, customer data, market data, and any other data that can provide insights into demand patterns. This data needs to be stored in a centralized database or data warehouse for easy access and analysis.
2. Data Cleaning and Preparation
Once the data is collected, it needs to be cleaned and prepared for analysis. This involves removing duplicates, correcting errors, and ensuring data consistency. Data normalization techniques may also be applied to ensure that data from different sources can be compared and analyzed effectively.
3. Data Analysis and Modeling
Once the data is cleaned and prepared, it is ready for analysis. Statistical algorithms and machine learning techniques are applied to the data to identify patterns, trends, and correlations. These insights are used to develop predictive models that can forecast future demand accurately.
4. Model Validation and Refinement
The developed predictive models need to be validated and refined to ensure their accuracy and reliability. This involves comparing the predicted results with actual sales data and making adjustments as necessary. Continuous monitoring and refinement of the models are essential to ensure their effectiveness over time.
5. Integration with Inventory Management Systems
Once the predictive models are validated and refined, they need to be integrated with the existing inventory management systems. This allows businesses to automate the inventory optimization process, making real-time adjustments to inventory levels based on the predictions. Integration with other supply chain systems, such as procurement and production, may also be necessary for seamless operations.
Conclusion
Predictive analytics plays a crucial role in optimizing inventory levels for logistics automation. By accurately forecasting demand, businesses can improve efficiency, reduce costs, enhance customer satisfaction, and make proactive decisions. Implementing predictive analytics requires collecting and integrating relevant data, cleaning and preparing the data, analyzing and modeling the data, validating and refining the models, and integrating them with inventory management systems. With the right implementation, businesses can achieve streamlined logistics, efficient inventory management, and improved overall supply chain performance.
Related Blogs
The benefits of implementing a preventive maintenance schedule for facility management
Read More