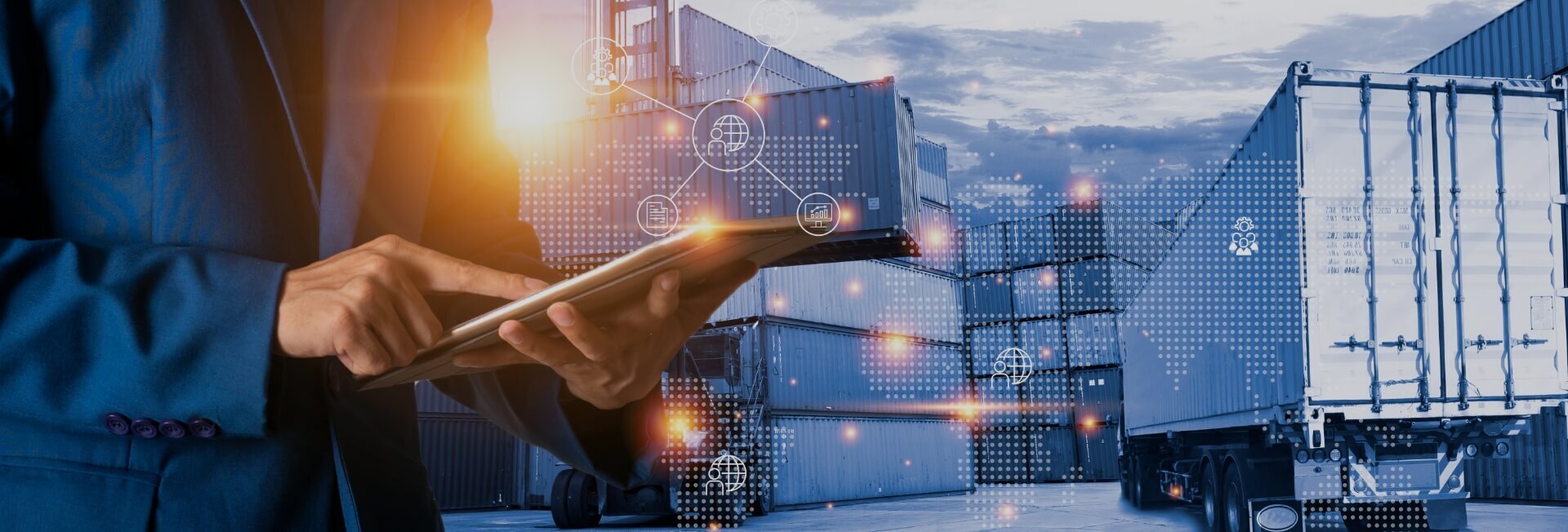
The role of predictive analytics in enhancing preventive maintenance strategies
- Home
- Resources
- Fleet Operations and Optimization
- The role of predictive analytics in enhancing preventive maintenance strategies
The Power of Predictive Analytics in Enhancing Preventive Maintenance Strategies
Preventive maintenance is a crucial aspect of fleet management and logistics operations. It involves regularly scheduled maintenance tasks that aim to prevent breakdowns, reduce downtime, and extend the lifespan of vehicles and equipment. Traditionally, preventive maintenance strategies rely on fixed time or usage-based schedules. However, with the advent of predictive analytics, businesses can now optimize their preventive maintenance activities by leveraging real-time data and actionable insights.
What is Predictive Analytics?
Predictive analytics is the practice of extracting information from historical data sets to identify patterns, trends, and relationships. By analyzing past data, predictive analytics algorithms can make predictions and generate actionable insights for future events or outcomes. In the context of preventive maintenance, predictive analytics enables businesses to anticipate equipment failures, identify maintenance needs, and schedule repairs proactively.
The Benefits of Predictive Analytics in Preventive Maintenance
Predictive analytics offers several benefits for enhancing preventive maintenance strategies in fleet management and logistics:
1. Improved Maintenance Scheduling
Predictive analytics allows businesses to move away from fixed time or usage-based maintenance schedules and adopt a more data-driven approach. By analyzing real-time fleet data, businesses can identify patterns and anomalies that indicate potential maintenance needs. This enables them to schedule maintenance activities at the right time, minimizing downtime and reducing the likelihood of unexpected breakdowns.
2. Enhanced Fleet Efficiency
By leveraging predictive analytics, businesses can optimize their fleet maintenance processes and improve overall efficiency. Real-time data on vehicle performance, fuel consumption, and maintenance requirements can be analyzed to identify areas of improvement and implement proactive measures. For example, predictive analytics can help identify inefficient vehicles that may require replacement or repairs, resulting in cost savings and streamlined operations.
3. Streamlined Logistics Operations
Predictive analytics can also play a significant role in optimizing logistics operations. By analyzing data on routes, traffic patterns, and maintenance needs, businesses can make informed decisions to streamline their supply chain and improve delivery times. For example, predictive analytics can help identify the most efficient routes, optimize load distribution, and schedule maintenance activities in a way that minimizes disruptions to the overall logistics process.
4. Cost Savings
Implementing predictive analytics in preventive maintenance strategies can result in significant cost savings for businesses. By proactively identifying maintenance needs and scheduling repairs before a breakdown occurs, businesses can avoid costly emergency repairs and reduce downtime. Additionally, predictive analytics can help optimize inventory management by ensuring that the right spare parts and supplies are available when needed, reducing unnecessary inventory holding costs.
How Predictive Analytics Works in Preventive Maintenance
Predictive analytics in preventive maintenance involves several key steps:
1. Data Collection
The first step in implementing predictive analytics is to collect relevant data from various sources. This can include data from onboard sensors, telematics systems, maintenance records, and historical performance data. The more comprehensive and accurate the data, the better the predictions and insights generated by the predictive analytics algorithms.
2. Data Cleaning and Preparation
Once the data is collected, it needs to be cleaned and prepared for analysis. This involves removing any outliers or inconsistencies, normalizing the data, and transforming it into a format that can be easily processed by the predictive analytics algorithms.
3. Data Analysis and Modeling
The next step is to analyze the data and develop predictive models. This involves applying statistical and machine learning techniques to identify patterns and relationships within the data. The predictive models are then trained using historical data to make accurate predictions and generate actionable insights.
4. Prediction and Actionable Insights
Once the predictive models are developed, they can be used to make predictions and generate actionable insights. For example, the models can predict when a particular vehicle is likely to experience a breakdown based on its historical performance data. This information can then be used to schedule maintenance activities proactively and prevent any potential issues.
5. Integration with Maintenance Management Systems
The final step is to integrate the predictive analytics insights with existing maintenance management systems. This ensures that the maintenance activities are scheduled and executed seamlessly. By automating the maintenance scheduling process, businesses can further improve efficiency and reduce the risk of human error.
Conclusion
Predictive analytics has revolutionized preventive maintenance strategies in fleet management and logistics. By leveraging real-time data and actionable insights, businesses can optimize their maintenance scheduling, improve fleet efficiency, streamline logistics operations, and achieve cost savings. Implementing predictive analytics in preventive maintenance allows businesses to move away from fixed schedules and adopt a more data-driven approach, resulting in comprehensive fleet management and enhanced logistics efficiency.
Related Blogs
The benefits of implementing a preventive maintenance schedule for facility management
Read More