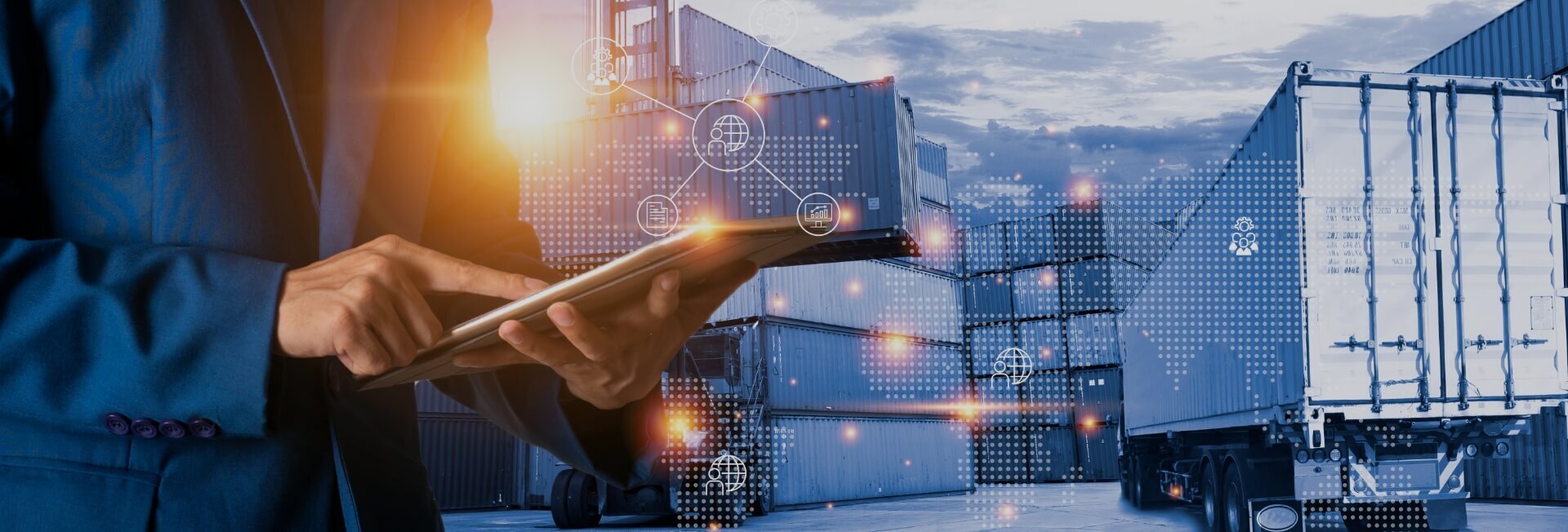
The role of predictive analytics in demand forecasting for logistics
- Home
- Resources
- Fleet Operations and Optimization
- The role of predictive analytics in demand forecasting for logistics
The Power of Predictive Analytics in Demand Forecasting for Logistics
In today's fast-paced world of logistics, efficient supply chain management is crucial to meet customer demands and stay ahead of the competition. One key aspect of supply chain management is demand forecasting, which involves predicting future demand for products or services. Traditionally, demand forecasting relied on historical data and statistical models. However, with the advent of predictive analytics, logistics companies now have a powerful tool to improve their demand forecasting accuracy and make informed business decisions. Let's explore the role of predictive analytics in demand forecasting for logistics and the benefits it brings to the table.
What is Predictive Analytics?
Predictive analytics is the practice of extracting actionable insights from data to predict future outcomes. It uses various statistical algorithms and machine learning techniques to analyze historical and real-time data, identify patterns, and make predictions. In the context of demand forecasting for logistics, predictive analytics enables companies to anticipate customer demands, optimize inventory levels, and streamline their supply chain operations.
Benefits of Predictive Analytics in Demand Forecasting
Incorporating predictive analytics into demand forecasting for logistics offers several key benefits, including:
1. Improved Accuracy
Predictive analytics leverages advanced algorithms and machine learning models to analyze vast amounts of data, including historical sales data, customer behavior, market trends, and external factors such as weather and holidays. By considering multiple variables and their correlations, predictive analytics can provide more accurate demand forecasts compared to traditional methods. This improved accuracy helps logistics companies optimize their inventory levels, reduce stockouts, and avoid excess inventory, leading to cost savings and improved customer satisfaction.
2. Real-Time Decision-Making
Traditional demand forecasting methods often rely on historical data, which may not capture real-time changes in customer behavior or market dynamics. Predictive analytics, on the other hand, can continuously analyze real-time data, allowing logistics companies to make more informed and timely decisions. For example, if a sudden increase in demand is detected, predictive analytics can alert the company to adjust their production or distribution plans accordingly, ensuring they can meet customer demands efficiently.
3. Enhanced Supply Chain Visibility
Predictive analytics provides logistics companies with greater visibility into their supply chain operations. By analyzing data from various sources, such as transportation systems, warehouse management systems, and customer relationship management systems, predictive analytics can identify bottlenecks, inefficiencies, and areas for improvement. This insight allows companies to optimize their supply chain processes, reduce lead times, and improve overall operational efficiency.
4. Streamlined Logistics and Maintenance Scheduling
Predictive analytics can also play a crucial role in optimizing logistics and maintenance scheduling for fleet management. By analyzing real-time fleet data, including vehicle performance, maintenance history, and environmental factors, predictive analytics can identify potential maintenance issues before they become major problems. This proactive approach to fleet maintenance not only minimizes vehicle breakdowns and repairs but also improves overall fleet efficiency and reduces operational costs.
The Future of Demand Forecasting in Logistics
As technology continues to advance, the role of predictive analytics in demand forecasting for logistics will only become more prominent. With the advent of the Internet of Things (IoT) and the availability of real-time data from various sources, logistics companies can leverage predictive analytics to gain even deeper insights into customer behavior, market trends, and supply chain dynamics. This will enable them to make more accurate demand forecasts, optimize inventory levels, and drive overall business growth.
Furthermore, the integration of predictive analytics with other emerging technologies, such as artificial intelligence and machine learning, will further enhance the capabilities of demand forecasting in logistics. These technologies can automate the demand forecasting process, continuously learn from data patterns, and adapt to changing market conditions. This will enable logistics companies to make data-driven decisions in real-time, optimize their supply chain operations, and stay ahead of the competition.
Conclusion
In conclusion, predictive analytics has revolutionized demand forecasting for logistics by providing accurate insights, real-time decision-making capabilities, enhanced supply chain visibility, and streamlined logistics and maintenance scheduling. By harnessing the power of predictive analytics, logistics companies can optimize their supply chain operations, reduce costs, improve customer satisfaction, and gain a competitive edge in the market. As technology continues to advance, the role of predictive analytics in demand forecasting will only become more vital for the success of logistics companies.
Related Blogs
The benefits of implementing a preventive maintenance schedule for facility management
Read More