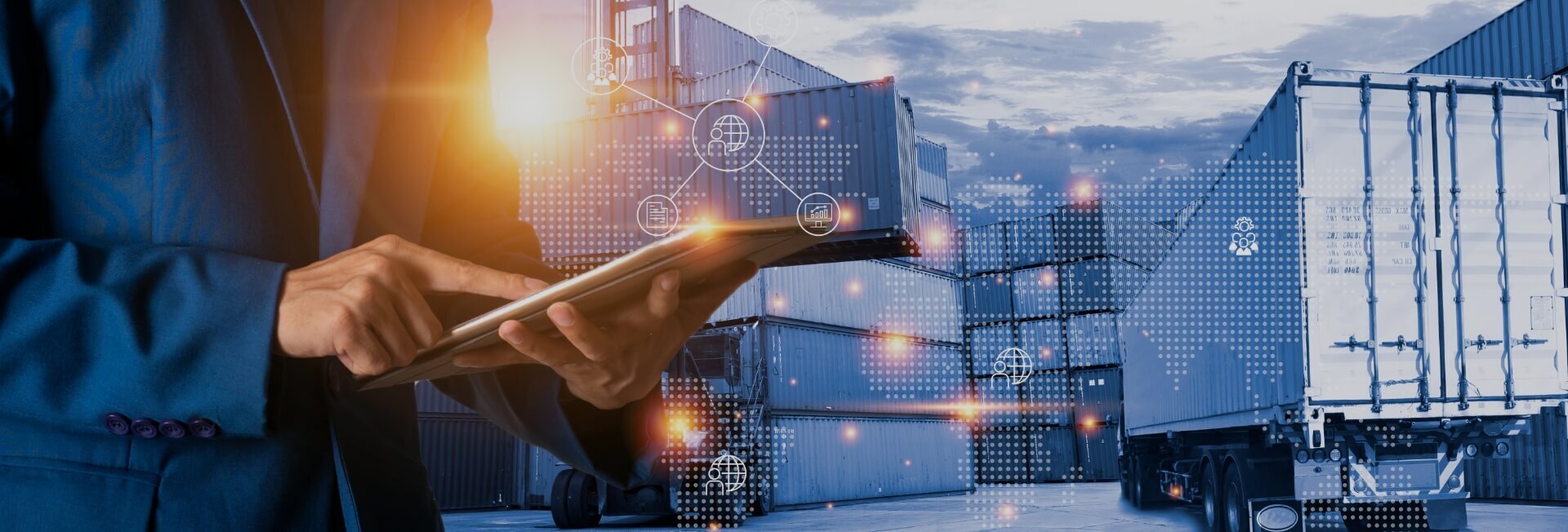
The role of machine learning in optimizing maintenance schedules
- Home
- Resources
- Fleet Operations and Optimization
- The role of machine learning in optimizing maintenance schedules
The Power of Machine Learning in Optimizing Maintenance Schedules
Preventive maintenance is crucial for fleet owners and operators to ensure the longevity and efficiency of their vehicles. Traditionally, maintenance schedules have been based on fixed intervals or mileage thresholds, but these approaches may not always be the most effective. With the advancements in technology, particularly in the field of machine learning, fleet maintenance can now be optimized to minimize downtime, reduce costs, and improve overall operational efficiency.
What is Machine Learning?
Machine learning is a subset of artificial intelligence that allows computers to learn and make predictions or take actions without being explicitly programmed. It involves the use of algorithms that analyze and interpret data to identify patterns or make informed decisions. In the context of fleet maintenance, machine learning algorithms can analyze real-time and historical data from vehicles to predict potential failures, identify optimal maintenance intervals, and provide actionable insights for fleet managers.
The Benefits of Optimized Maintenance Schedules
Optimizing maintenance schedules using machine learning can bring several benefits to fleet owners and operators:
1. Minimized Downtime and Repairs
By analyzing real-time data from vehicles, machine learning algorithms can detect early signs of potential failures or malfunctions. This allows fleet managers to proactively schedule maintenance or repairs before the issue becomes critical, minimizing downtime and preventing costly breakdowns. With optimized maintenance schedules, vehicles are less likely to experience unexpected failures, resulting in improved operational efficiency and customer satisfaction.
2. Reduced Maintenance Costs
Traditional maintenance schedules often rely on fixed intervals or mileage thresholds, which may lead to unnecessary maintenance or replacement of parts that are still in good condition. Machine learning algorithms can analyze data from individual vehicles, taking into account factors such as usage patterns, driving conditions, and vehicle health, to determine the optimal maintenance intervals for each specific vehicle. By avoiding unnecessary maintenance, fleet owners can significantly reduce maintenance costs.
3. Improved Safety and Compliance
Maintaining a fleet of vehicles in top condition is essential for ensuring the safety of drivers, passengers, and other road users. Machine learning algorithms can analyze data from various sensors and monitoring systems in vehicles to detect potential safety issues, such as brake wear, tire pressure, or engine malfunctions. By identifying these issues early on, fleet managers can take immediate action to address them, ensuring compliance with safety regulations and reducing the risk of accidents.
4. Enhanced Fuel Efficiency
Optimized maintenance schedules can also contribute to improved fuel efficiency. Machine learning algorithms can analyze data from vehicles, such as fuel consumption rates, engine performance, and driving patterns, to identify areas for improvement. By addressing maintenance issues or optimizing driving techniques, fleet managers can reduce fuel consumption and lower overall operating costs.
The Role of Real-Time Fleet Data
Real-time fleet data plays a crucial role in optimizing maintenance schedules using machine learning. Fleet management solutions equipped with telematics devices can gather and transmit data from vehicles to a centralized platform. This data includes information such as vehicle location, engine performance, fuel consumption, and diagnostic codes.
Machine learning algorithms can analyze this real-time data and compare it with historical data to identify patterns and potential issues. For example, if a particular vehicle is consistently experiencing higher than average fuel consumption, the algorithm can identify the possible causes and recommend maintenance actions or driver training to address the issue.
Implementing Machine Learning for Maintenance Optimization
Implementing machine learning for maintenance optimization requires a comprehensive fleet management solution that integrates with telematics devices and provides advanced analytics capabilities. Here are the key steps involved:
1. Data Collection and Integration
The first step is to collect and integrate data from various sources, including telematics devices, sensors, and maintenance records. This data should be standardized and aggregated in a centralized platform for analysis.
2. Data Analysis and Modeling
Once the data is collected, it needs to be analyzed and modeled using machine learning algorithms. This involves identifying relevant variables, defining maintenance intervals, and creating predictive models based on historical and real-time data.
3. Predictive Maintenance Recommendations
Based on the analysis and modeling, the machine learning algorithms can generate predictive maintenance recommendations for each individual vehicle in the fleet. These recommendations can include specific maintenance actions, optimal scheduling, and estimated costs.
4. Integration with Maintenance Workflow
The final step is to integrate the machine learning recommendations with the existing maintenance workflow. This can involve notifying maintenance technicians, scheduling maintenance appointments, and tracking the completion of recommended actions.
The Future of Maintenance Optimization
The role of machine learning in optimizing maintenance schedules is continually evolving. As technology advances and more data becomes available, machine learning algorithms will become even more sophisticated in their ability to predict failures and optimize maintenance intervals.
Furthermore, the integration of machine learning with other technologies, such as Internet of Things (IoT) devices and predictive analytics, will enable even more comprehensive and proactive maintenance strategies. For example, IoT sensors can continuously monitor the health of various vehicle components and transmit real-time data to machine learning algorithms for analysis and prediction of potential failures.
In conclusion, machine learning has the potential to revolutionize fleet maintenance by optimizing maintenance schedules and providing actionable insights. By leveraging real-time and historical data, fleet owners and operators can minimize downtime, reduce costs, and improve overall operational efficiency. As technology continues to advance, the role of machine learning in maintenance optimization will only become more significant, enabling fleet owners to stay ahead in an increasingly competitive industry.
Related Blogs
The benefits of implementing a preventive maintenance schedule for facility management
Read More