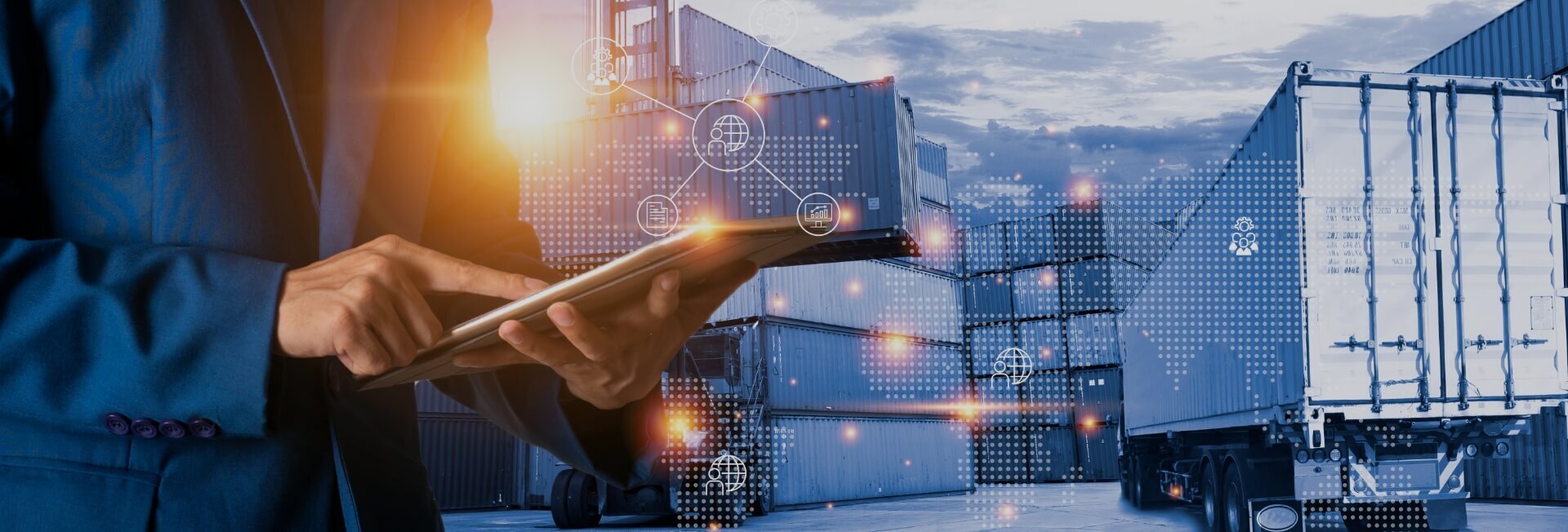
The role of machine learning in optimizing logistics routing for improved efficiency
- Home
- Resources
- Fleet Operations and Optimization
- The role of machine learning in optimizing logistics routing for improved efficiency
The Role of Machine Learning in Optimizing Logistics Routing for Improved Efficiency
Logistics routing plays a crucial role in the transportation industry, ensuring that goods are delivered efficiently and on time. Traditionally, this process has relied on manual planning and decision-making, which can be time-consuming and prone to errors. However, with the advent of machine learning, logistics routing is undergoing a significant transformation. Machine learning algorithms are revolutionizing the way businesses optimize their supply chain, enhance fleet maintenance, and make real-time decisions. In this article, we will explore the role of machine learning in optimizing logistics routing for improved efficiency.
1. Fleet Maintenance Optimization
Fleet maintenance is a critical aspect of logistics operations. Regular maintenance and timely repairs are essential to ensure the smooth functioning of vehicles and prevent unexpected breakdowns. Machine learning algorithms can analyze real-time fleet data, identify patterns, and provide actionable insights to optimize maintenance scheduling.
By leveraging machine learning, logistics companies can predict maintenance requirements and proactively schedule repairs, reducing the risk of unplanned downtime. This preventive maintenance approach not only enhances fleet efficiency but also minimizes repair costs and extends the lifespan of vehicles. Machine learning algorithms can analyze historical data, such as vehicle usage patterns, maintenance records, and environmental conditions, to generate accurate maintenance schedules tailored to each vehicle's specific needs.
2. Supply Chain Optimization
Efficient supply chain management is crucial for businesses to remain competitive in today's fast-paced market. Machine learning algorithms can optimize supply chain processes by providing real-time visibility and actionable insights. With the ability to analyze vast amounts of data, including customer demand, inventory levels, and transportation costs, machine learning algorithms can identify bottlenecks, streamline operations, and improve overall supply chain efficiency.
By integrating machine learning into logistics routing, businesses can achieve end-to-end visibility of their supply chain. Real-time data analysis enables companies to make informed decisions, such as rerouting shipments to avoid congestion or optimizing delivery routes to minimize fuel consumption. This data-driven approach to supply chain optimization can result in significant cost savings, improved customer satisfaction, and enhanced operational efficiency.
3. Real-Time Decision-Making
In the logistics industry, time is of the essence. Real-time decision-making is crucial for ensuring on-time deliveries, managing unexpected events, and responding to changing customer demands. Machine learning algorithms can process real-time data from various sources, such as GPS trackers, weather forecasts, and traffic information, to make informed decisions on the fly.
For example, in the event of a traffic jam or road closure, machine learning algorithms can quickly analyze alternative routes and provide drivers with real-time instructions to avoid delays. By leveraging machine learning, logistics companies can optimize their routing strategies and adapt to changing circumstances, resulting in faster and more efficient deliveries.
4. Streamlined Logistics Operations
Machine learning algorithms can streamline logistics operations by automating repetitive tasks and reducing manual intervention. For example, machine learning can automate the process of assigning vehicles to deliveries based on factors such as distance, capacity, and delivery time. By automating these tasks, logistics companies can reduce human error, optimize resource allocation, and improve overall operational efficiency.
In addition to automating routine tasks, machine learning algorithms can also optimize complex decision-making processes. For example, when multiple shipments need to be consolidated into a single route, machine learning algorithms can analyze various factors, such as delivery deadlines, vehicle capacity, and traffic conditions, to determine the most efficient route and load distribution.
Conclusion
Machine learning is revolutionizing logistics routing, enabling businesses to optimize fleet maintenance, streamline supply chain operations, make real-time decisions, and automate repetitive tasks. By leveraging machine learning algorithms, logistics companies can achieve comprehensive fleet management, improve maintenance scheduling, enhance supply chain visibility, and increase overall operational efficiency. As the technology continues to advance, we can expect further innovations in logistics automation and data-driven decision-making, ultimately leading to more efficient and sustainable logistics operations.
Related Blogs
The benefits of implementing a preventive maintenance schedule for facility management
Read More