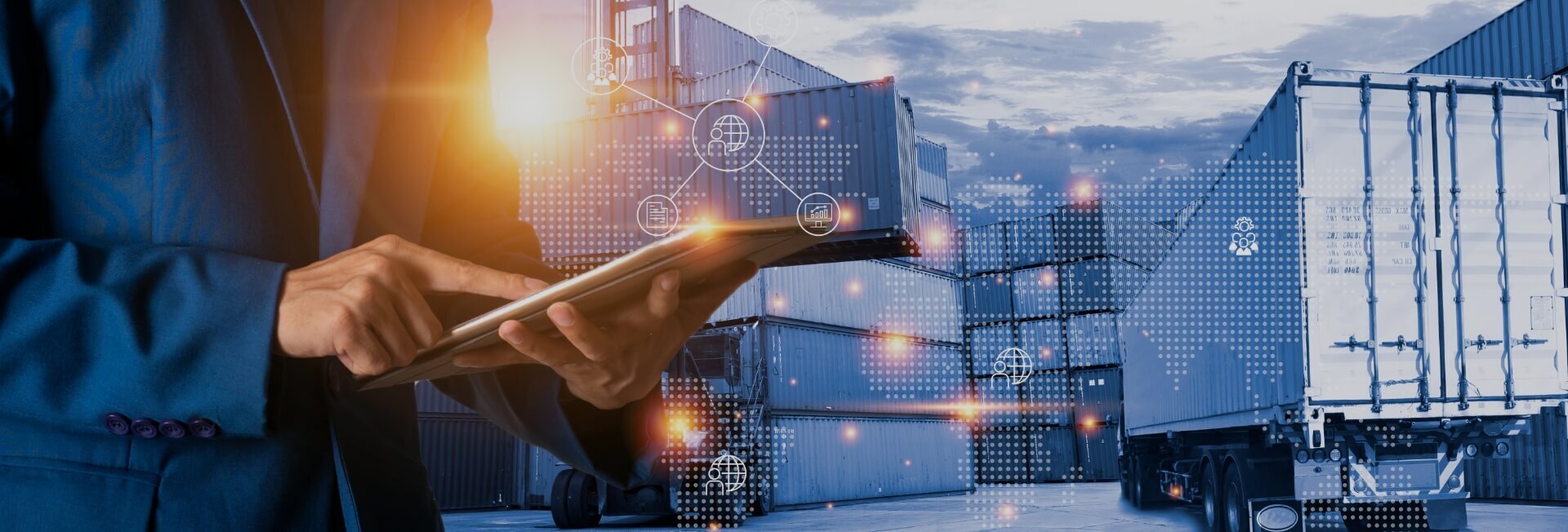
The role of machine learning in optimizing logistics processes
- Home
- Resources
- Fleet Operations and Optimization
- The role of machine learning in optimizing logistics processes
The Role of Machine Learning in Optimizing Logistics Processes
The logistics industry has always been focused on improving efficiency and reducing costs. With the advent of machine learning, logistics companies now have the tools to optimize their processes and achieve new levels of efficiency. Machine learning algorithms can analyze vast amounts of data, identify patterns, and generate actionable insights that can streamline logistics operations, improve fleet maintenance, and enhance supply chain visibility.
Streamlined Logistics with Machine Learning
One of the key benefits of machine learning in logistics is the ability to streamline operations. By analyzing historical data, machine learning algorithms can identify inefficiencies in the supply chain and suggest optimizations. For example, machine learning can analyze delivery routes and recommend the most efficient routes based on factors such as traffic patterns, weather conditions, and delivery time windows. This not only reduces transit time but also minimizes fuel consumption and carbon emissions.
Furthermore, machine learning can optimize inventory management by analyzing demand patterns and predicting future demand. By accurately forecasting demand, logistics companies can optimize their inventory levels, reducing the risk of stockouts or excess inventory. This leads to improved customer satisfaction, reduced storage costs, and increased overall efficiency.
Optimizing Fleet Maintenance with Machine Learning
Fleet maintenance is a critical aspect of logistics operations. Downtime due to vehicle breakdowns can disrupt supply chains, delay deliveries, and result in additional costs. Machine learning can play a crucial role in optimizing fleet maintenance by enabling preventive maintenance and predicting equipment failures.
By analyzing real-time fleet data, machine learning algorithms can identify patterns that indicate potential equipment failures. For example, if a certain combination of parameters such as engine temperature, oil pressure, and mileage is associated with a past breakdown, the algorithm can identify similar patterns in real-time data and predict an upcoming failure. This allows fleet managers to proactively schedule maintenance, preventing breakdowns and minimizing downtime.
Machine learning can also optimize maintenance scheduling by considering multiple factors such as vehicle availability, maintenance crew availability, and delivery schedules. By analyzing historical data, the algorithm can recommend the most optimal maintenance schedule that minimizes the impact on operations and maximizes fleet availability.
Enhancing Supply Chain Visibility and Optimization
Supply chain visibility is crucial for efficient logistics operations. Machine learning algorithms can analyze real-time data from various sources, such as GPS tracking, warehouse inventory systems, and customer order data, to provide comprehensive visibility into the supply chain.
By integrating data from different sources, machine learning algorithms can identify bottlenecks, optimize routing, and improve delivery accuracy. For example, if the algorithm detects a delay in a certain shipment, it can automatically reroute other shipments to avoid further delays. This ensures that deliveries are made on time and customer satisfaction is maintained.
Machine learning can also optimize supply chain planning by considering multiple factors such as demand forecasts, inventory levels, production capacities, and transportation constraints. By simulating different scenarios and analyzing historical data, the algorithm can generate optimized plans that minimize costs and maximize efficiency.
Data-Driven Decision-Making in Logistics
Machine learning enables data-driven decision-making in logistics. By analyzing vast amounts of data, machine learning algorithms can generate actionable insights that aid in real-time decision-making. For example, if the algorithm detects a sudden increase in demand for a certain product, it can recommend adjusting production schedules or reallocating inventory to meet the demand.
Furthermore, machine learning algorithms can continuously learn and improve over time. By analyzing the outcomes of previous decisions and incorporating feedback, the algorithms can refine their models and improve their accuracy. This iterative learning process enables logistics companies to constantly optimize their operations and stay ahead of the competition.
The Future of Logistics with Machine Learning
Machine learning is revolutionizing the logistics industry by optimizing processes, improving fleet maintenance, and enhancing supply chain visibility. As technology continues to evolve, we can expect even more advanced machine learning algorithms that can analyze complex data sets and generate insights in real-time.
With the increasing availability of Internet of Things (IoT) devices and sensors, logistics companies will have access to even more real-time data. This data can be used to further optimize logistics operations, improve fleet efficiency, and enhance customer service.
Machine learning will also play a crucial role in the automation of logistics processes. By leveraging machine learning algorithms, logistics companies can automate tasks such as route planning, inventory management, and demand forecasting. This not only improves efficiency but also reduces the risk of human error.
In conclusion, machine learning is transforming the logistics industry by optimizing processes, improving fleet maintenance, enhancing supply chain visibility, and enabling data-driven decision-making. As logistics companies continue to embrace machine learning technologies, they will be able to achieve comprehensive fleet management, efficient logistics operations, and real-time decision-making. The future of logistics is data-driven, efficient, and optimized with the help of machine learning.
Related Blogs
The benefits of implementing a preventive maintenance schedule for facility management
Read More