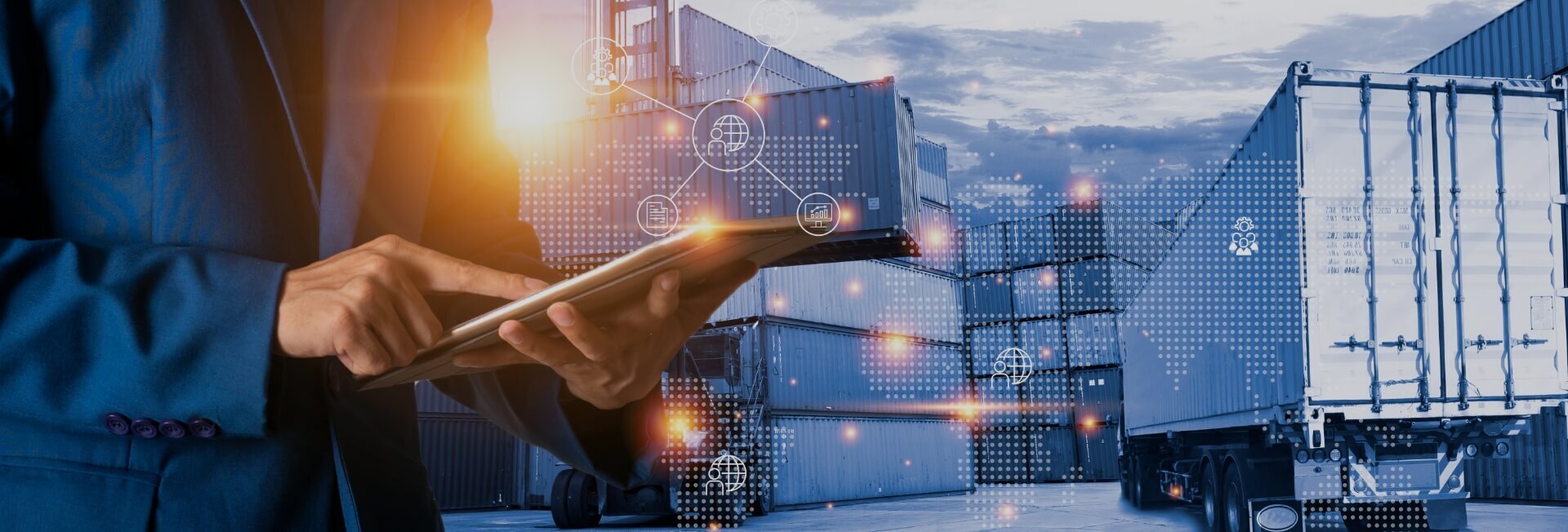
The role of machine learning in improving fleet automation systems
- Home
- Resources
- Fleet Operations and Optimization
- The role of machine learning in improving fleet automation systems
Introduction
Fleet automation systems have revolutionized the way logistics and transportation companies manage their fleets. With the help of advanced technologies such as machine learning, these systems have become more efficient, reliable, and cost-effective. Machine learning algorithms can analyze vast amounts of data in real-time, enabling fleet managers to make data-driven decisions and optimize their operations. In this article, we will explore the role of machine learning in improving fleet automation systems and the benefits it brings to the logistics industry.
Improving Preventive Maintenance
Preventive maintenance is crucial for keeping a fleet of vehicles in optimal condition and reducing the risk of unexpected breakdowns. Machine learning algorithms can analyze historical maintenance data, including vehicle usage, repair history, and environmental factors, to identify patterns and predict when maintenance is required. By implementing predictive maintenance strategies based on machine learning insights, fleet managers can schedule maintenance proactively, minimizing downtime and reducing maintenance costs.
Enhancing Fleet Tracking and Supply Chain Visibility
Real-time tracking of fleet vehicles is essential for efficient logistics and supply chain management. Machine learning algorithms can analyze GPS and sensor data from vehicles to provide accurate and up-to-date location information. This enables fleet managers to track the movement of vehicles in real-time, optimize routes, and respond quickly to unforeseen events such as traffic congestion or accidents. Additionally, machine learning can help improve supply chain visibility by integrating data from various sources, such as suppliers, warehouses, and retailers, to provide a comprehensive view of the entire supply chain. This allows for better planning, coordination, and optimization of logistics operations.
Optimizing Maintenance Scheduling
Maintenance scheduling is a critical aspect of fleet management. Machine learning algorithms can analyze real-time data from vehicles, such as engine performance, fuel consumption, and mileage, to determine the optimal time for maintenance. By considering various factors, such as vehicle workload, maintenance history, and operational requirements, machine learning can generate accurate and personalized maintenance schedules for each vehicle in the fleet. This ensures that maintenance tasks are performed at the right time, reducing the risk of breakdowns, improving fleet performance, and extending the lifespan of vehicles.
Streamlining Vehicle Repairs
When a vehicle requires repair, it is essential to diagnose the problem accurately and determine the most efficient and cost-effective solution. Machine learning algorithms can analyze sensor data from vehicles to detect anomalies and predict potential failures. By comparing the current sensor readings with historical data, machine learning algorithms can identify patterns and anomalies that indicate potential issues. This allows fleet managers to address problems proactively, schedule repairs in advance, and minimize vehicle downtime. Additionally, machine learning can help optimize repair processes by recommending the most suitable repair facilities, parts, and technicians based on historical data and performance metrics.
Improving Fleet Optimization and Efficiency
Machine learning plays a significant role in optimizing fleet operations and improving efficiency. By analyzing historical data on vehicle usage, fuel consumption, driver behavior, and other relevant factors, machine learning algorithms can identify areas of improvement and suggest actionable insights. For example, machine learning can identify inefficient routes, excessive idling, or aggressive driving behavior that leads to increased fuel consumption and higher maintenance costs. By providing recommendations and real-time feedback to drivers and fleet managers, machine learning algorithms can help optimize fleet operations, reduce fuel consumption, and improve overall efficiency.
Enabling Data-Driven Fleet Management
Machine learning enables fleet managers to make data-driven decisions by providing real-time insights and predictive analytics. By analyzing large volumes of data, including vehicle diagnostics, maintenance records, driver performance, and external factors such as weather and traffic conditions, machine learning algorithms can identify patterns and correlations that human operators may overlook. This allows fleet managers to identify areas for improvement, prioritize resources, and make informed decisions that maximize fleet performance and profitability.
Conclusion
Machine learning is revolutionizing fleet automation systems by enabling proactive maintenance, enhancing fleet tracking and supply chain visibility, optimizing maintenance scheduling, streamlining vehicle repairs, improving fleet optimization and efficiency, and enabling data-driven fleet management. By leveraging the power of machine learning algorithms, logistics and transportation companies can achieve comprehensive fleet management, reduce costs, improve customer service, and gain a competitive edge in the industry. As technology continues to advance, the role of machine learning in fleet automation systems will only become more critical, shaping the future of logistics and transportation.
Related Blogs
The benefits of implementing a preventive maintenance schedule for facility management
Read More