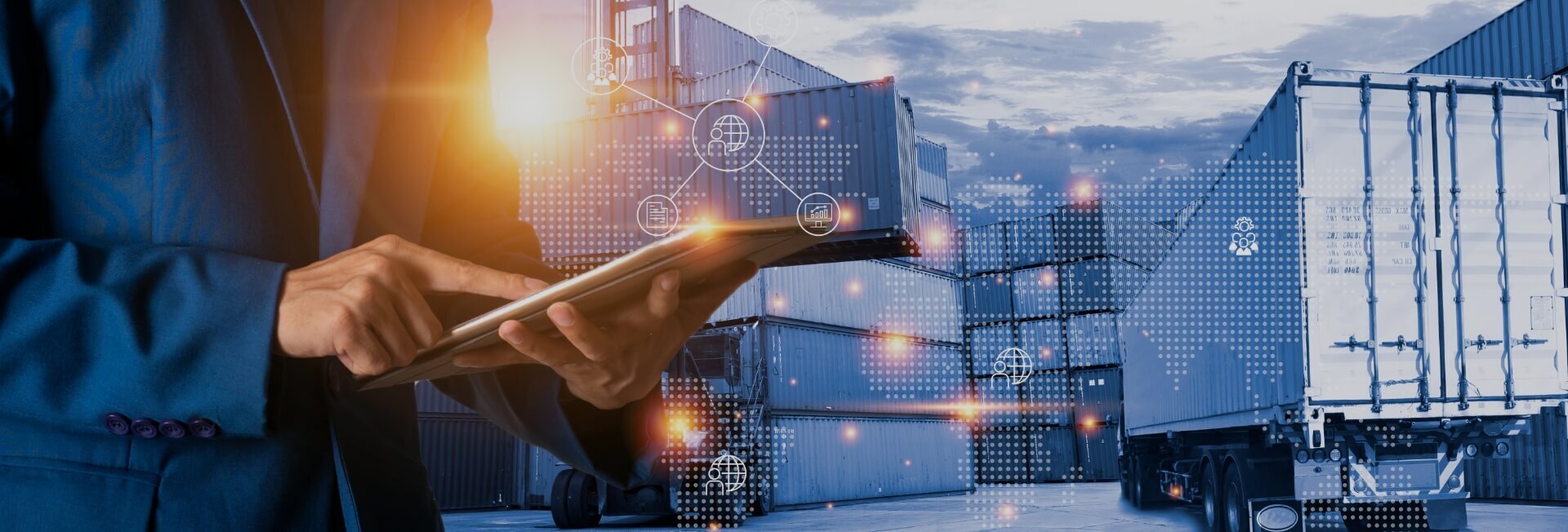
The role of artificial neural networks in predictive maintenance automation
- Home
- Resources
- Fleet Operations and Optimization
- The role of artificial neural networks in predictive maintenance automation
The Future of Predictive Maintenance Automation: Artificial Neural Networks
In today's fast-paced world, businesses rely heavily on efficient logistics and fleet management to ensure smooth operations. The ability to predict and prevent maintenance issues before they occur is crucial for minimizing downtime, reducing costs, and improving overall fleet efficiency. This is where artificial neural networks (ANNs) come into play. ANNs have revolutionized the field of predictive maintenance automation, enabling businesses to streamline their operations and make data-driven decisions.
What is Predictive Maintenance Automation?
Predictive maintenance automation is the process of using advanced technologies and data analytics to predict when and where maintenance issues are likely to occur in a fleet of vehicles or machinery. By analyzing real-time data, historical patterns, and other relevant factors, businesses can proactively schedule maintenance tasks, optimize their resources, and prevent costly breakdowns. This approach is far more efficient and cost-effective compared to traditional preventive maintenance, where maintenance tasks are performed based on a predefined schedule.
The Role of Artificial Neural Networks in Predictive Maintenance Automation
Artificial neural networks play a crucial role in predictive maintenance automation. ANNs are a type of machine learning algorithm inspired by the structure and functioning of the human brain. They are designed to recognize patterns, learn from data, and make predictions or decisions based on that learning. In the context of predictive maintenance, ANNs can analyze large volumes of data collected from sensors, maintenance logs, and other sources to identify patterns and anomalies that may indicate a potential maintenance issue.
By training ANNs on historical maintenance data, businesses can create models that can accurately predict when a particular component or system is likely to fail. These predictions can then be used to trigger maintenance alerts, generate work orders, and schedule repairs or replacements. This proactive approach helps businesses avoid costly breakdowns, minimize downtime, and optimize their maintenance resources.
Benefits of Artificial Neural Networks in Predictive Maintenance Automation
There are several benefits of using artificial neural networks in predictive maintenance automation:
1. Improved Fleet Maintenance Efficiency
By accurately predicting maintenance issues, ANNs allow businesses to proactively schedule repairs and replacements. This reduces the chances of unexpected breakdowns and minimizes downtime. With fewer unplanned maintenance tasks, businesses can optimize their resources and improve overall fleet maintenance efficiency.
2. Cost Savings
Predictive maintenance automation helps businesses save costs in multiple ways. By preventing breakdowns and minimizing downtime, businesses can avoid expensive repairs and reduce the need for emergency maintenance services. Additionally, by optimizing maintenance schedules and resource allocation, businesses can reduce unnecessary maintenance tasks and save on labor and parts costs.
3. Data-Driven Decision Making
Artificial neural networks enable businesses to make data-driven decisions when it comes to fleet maintenance. By analyzing real-time data and historical patterns, businesses can gain actionable insights into the health of their fleet and make informed decisions about maintenance tasks, repairs, and replacements. This helps businesses optimize their maintenance strategies and improve overall fleet performance.
4. Streamlined Logistics and Supply Chain Optimization
Predictive maintenance automation is closely linked to logistics and supply chain visibility. By accurately predicting maintenance issues, businesses can plan their logistics operations more effectively, ensuring that maintenance tasks do not disrupt the flow of goods and materials. This leads to streamlined logistics, improved supply chain visibility, and optimized supply chain operations.
The Future of Predictive Maintenance Automation
The future of predictive maintenance automation lies in the continued advancement of artificial neural networks and other machine learning techniques. As technology evolves, ANNs will become even more sophisticated in their ability to analyze complex data sets and make accurate predictions. This will further enhance the efficiency and effectiveness of predictive maintenance automation, allowing businesses to minimize downtime, reduce costs, and optimize their fleet operations.
Furthermore, the integration of ANNs with other emerging technologies, such as Internet of Things (IoT) devices and big data analytics, will enable businesses to collect and analyze real-time fleet data on a larger scale. This will provide even more comprehensive insights into the health of the fleet and enable businesses to take proactive measures to prevent maintenance issues.
In conclusion, artificial neural networks are revolutionizing the field of predictive maintenance automation. By leveraging the power of machine learning, businesses can accurately predict maintenance issues, optimize their resources, and make data-driven decisions. This leads to improved fleet maintenance efficiency, cost savings, streamlined logistics, and enhanced supply chain optimization. As technology continues to advance, the role of ANNs in predictive maintenance automation will only become more significant, paving the way for a future of efficient and proactive fleet management.
Related Blogs
The benefits of implementing a preventive maintenance schedule for facility management
Read More