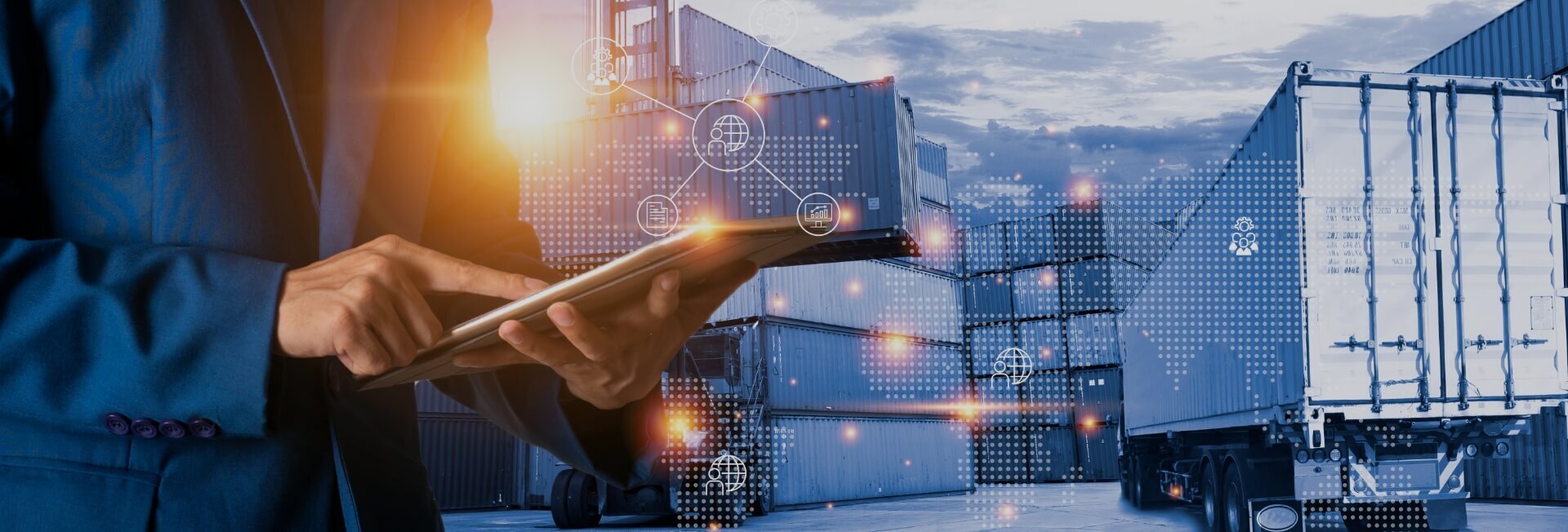
The benefits of using machine learning algorithms in route optimization for logistics automation
- Home
- Resources
- Fleet Operations and Optimization
- The benefits of using machine learning algorithms in route optimization for logistics automation
The Power of Machine Learning in Logistics Route Optimization
Logistics and supply chain management are critical to the success of any business that relies on the efficient movement of goods. In today's fast-paced and globalized world, companies are constantly seeking ways to streamline their logistics operations and gain a competitive edge. One area that has seen significant advancements in recent years is route optimization, and machine learning algorithms play a crucial role in this process.
What is Route Optimization?
Route optimization is the process of determining the most efficient route for a vehicle to take based on various factors such as distance, traffic conditions, delivery/pickup time windows, and vehicle capacity. Traditionally, this task was performed manually by logistics planners, who would use their experience and intuition to plan routes. However, with the advent of machine learning and advanced algorithms, route optimization has become much more automated and data-driven.
The Role of Machine Learning Algorithms in Route Optimization
Machine learning algorithms have the ability to analyze large amounts of data and identify patterns and trends that humans may not be able to detect. In the context of logistics route optimization, these algorithms can take into account real-time data such as traffic conditions, weather forecasts, and historical delivery data to make accurate predictions about the best routes to take.
One of the key benefits of using machine learning algorithms in route optimization is the ability to make real-time adjustments. For example, if a delivery truck encounters unexpected traffic congestion, the algorithm can quickly reroute the vehicle to minimize delays and ensure on-time delivery. This level of agility and adaptability is crucial in today's fast-paced business environment.
Benefits of Using Machine Learning Algorithms in Route Optimization
There are several benefits to using machine learning algorithms in route optimization for logistics automation:
1. Improved Efficiency
By optimizing routes, machine learning algorithms can significantly improve the efficiency of logistics operations. By minimizing travel distances and reducing idle time, companies can save on fuel costs and increase the number of deliveries they can make in a given time period. This leads to cost savings and improved customer satisfaction due to faster delivery times.
2. Enhanced Customer Service
Route optimization algorithms can also help improve customer service by providing accurate delivery time estimates and reducing the likelihood of missed or delayed deliveries. Customers appreciate reliable and punctual service, and by leveraging machine learning algorithms, companies can meet and exceed customer expectations.
3. Reduced Maintenance Costs
Machine learning algorithms can also contribute to preventive maintenance and fleet optimization. By analyzing real-time fleet data, these algorithms can detect potential issues before they become major problems, allowing companies to schedule maintenance and repairs proactively. This proactive approach can help reduce downtime and costly breakdowns, resulting in significant cost savings.
4. Data-Driven Decision Making
Machine learning algorithms provide actionable insights based on data analysis. By leveraging real-time data and historical trends, logistics planners can make informed decisions about route planning, resource allocation, and overall fleet management. This data-driven approach leads to more efficient and effective logistics operations.
Challenges of Implementing Machine Learning in Logistics Route Optimization
While the benefits of using machine learning algorithms in route optimization are clear, there are also challenges associated with implementing this technology:
1. Data Quality and Availability
Machine learning algorithms rely on high-quality and up-to-date data to make accurate predictions. However, in the logistics industry, data quality and availability can be a challenge. Companies need to ensure that they have access to reliable data sources and invest in data management and integration solutions to overcome this challenge.
2. Integration with Existing Systems
Integrating machine learning algorithms into existing logistics systems can be complex and time-consuming. Companies need to ensure that their IT infrastructure is capable of handling the additional computational requirements and that the algorithms can seamlessly integrate with other systems such as fleet management software and GPS tracking devices.
3. Skill and Knowledge Gap
Implementing machine learning algorithms requires specialized skills and knowledge. Companies need to invest in training their employees or hire data scientists and machine learning experts to ensure the successful implementation and utilization of these algorithms.
Conclusion
Machine learning algorithms have revolutionized logistics route optimization by enabling companies to make data-driven decisions and improve the efficiency of their operations. By leveraging real-time data and historical trends, these algorithms can optimize routes, reduce fuel costs, enhance customer service, and proactively manage fleet maintenance. While there are challenges associated with implementing this technology, the benefits far outweigh the costs. As the logistics industry continues to evolve, machine learning algorithms will play an increasingly important role in driving efficiency and streamlining operations.
Related Blogs
The benefits of implementing a preventive maintenance schedule for facility management
Read More