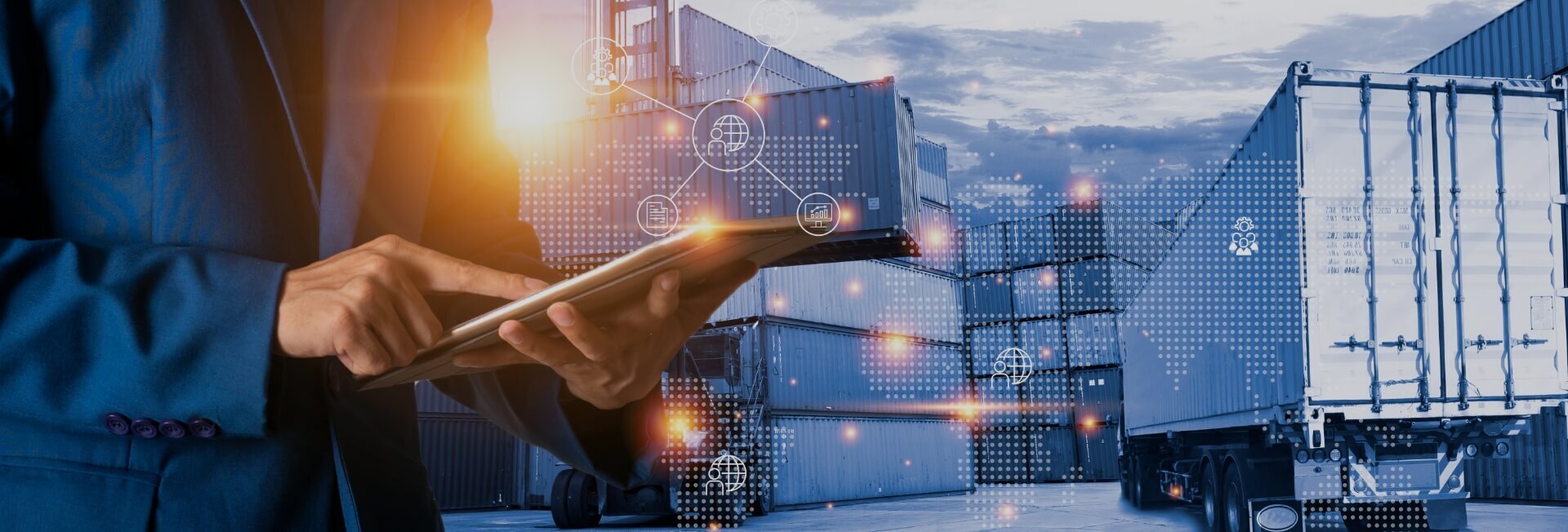
The benefits of using machine learning algorithms in demand forecasting for logistics automation
- Home
- Resources
- Fleet Operations and Optimization
- The benefits of using machine learning algorithms in demand forecasting for logistics automation
The Power of Machine Learning in Logistics Automation
Logistics automation has become an essential component of modern supply chain management. With the rise of e-commerce and the increasing customer expectations for fast and efficient delivery, businesses are constantly seeking ways to streamline their logistics operations and optimize their supply chains. One of the key technologies driving this transformation is machine learning. By leveraging machine learning algorithms, companies can gain valuable insights into demand patterns, improve forecast accuracy, and enhance overall logistics efficiency.
Enhanced Demand Forecasting
Accurate demand forecasting is crucial for logistics operations as it enables businesses to plan and allocate resources effectively. Traditional demand forecasting methods often rely on historical data and manual analysis, which can be time-consuming and prone to errors. Machine learning algorithms, on the other hand, can analyze large volumes of data from various sources, including historical sales data, customer behavior, weather patterns, and economic trends, to generate accurate and real-time demand forecasts.
By using machine learning algorithms, businesses can identify patterns and correlations in the data that humans may not be able to detect. This enables them to make more informed decisions regarding inventory management, production planning, and transportation optimization. With accurate demand forecasts, businesses can reduce stockouts, minimize excess inventory, and improve customer satisfaction by ensuring timely deliveries.
Optimized Supply Chain Management
Machine learning algorithms can also be applied to optimize various aspects of supply chain management, including inventory control, transportation planning, and warehouse management. By analyzing historical data and real-time information, machine learning algorithms can identify trends and patterns in customer demand, enabling businesses to optimize their inventory levels and reduce carrying costs.
Furthermore, machine learning can help businesses optimize their transportation routes and schedules. By considering factors such as traffic conditions, fuel prices, and delivery deadlines, machine learning algorithms can identify the most efficient routes and recommend optimal delivery schedules. This not only reduces transportation costs but also improves delivery speed and reliability.
Preventive Maintenance and Fleet Optimization
Maintenance plays a critical role in ensuring the smooth operation of logistics fleets. Unscheduled breakdowns and vehicle repairs can cause delays, disrupt supply chains, and increase costs. Machine learning algorithms can help businesses implement preventive maintenance strategies by analyzing real-time fleet data, identifying potential maintenance issues, and recommending proactive maintenance actions.
By leveraging machine learning algorithms, businesses can detect patterns and anomalies in fleet data, such as engine performance, fuel consumption, and tire wear. This allows them to predict when maintenance is required and schedule it in advance, minimizing the risk of unexpected breakdowns and reducing overall maintenance costs.
In addition to preventive maintenance, machine learning algorithms can also optimize fleet utilization and allocation. By analyzing historical data and real-time information, machine learning algorithms can identify underutilized assets, recommend optimal allocation strategies, and improve overall fleet efficiency. This not only reduces costs but also enhances logistics visibility and enables real-time decision-making.
Actionable Insights for Streamlined Logistics
One of the key advantages of using machine learning algorithms in logistics automation is the ability to generate actionable insights. By analyzing large volumes of data, machine learning algorithms can identify trends, patterns, and correlations that humans may not be able to detect. These insights can help businesses make more informed decisions and take proactive actions to streamline their logistics operations.
For example, machine learning algorithms can analyze customer behavior data to identify purchasing patterns and preferences. This can help businesses optimize their product assortment and pricing strategies to meet customer demands and increase sales. Machine learning algorithms can also analyze transportation data to identify bottlenecks and inefficiencies in the supply chain, enabling businesses to implement process improvements and optimize their logistics networks.
Conclusion
Machine learning algorithms offer immense potential for enhancing demand forecasting, optimizing supply chain management, improving maintenance scheduling, and generating actionable insights in logistics automation. By leveraging the power of machine learning, businesses can improve forecast accuracy, reduce costs, enhance customer satisfaction, and gain a competitive edge in today's fast-paced and highly competitive business environment.
As logistics operations continue to evolve and become more complex, the use of machine learning algorithms will become increasingly critical for businesses that want to stay ahead of the curve. By embracing machine learning and leveraging its capabilities, businesses can unlock the full potential of their data and drive efficiency and innovation in their logistics operations.
Related Blogs
The benefits of implementing a preventive maintenance schedule for facility management
Read More