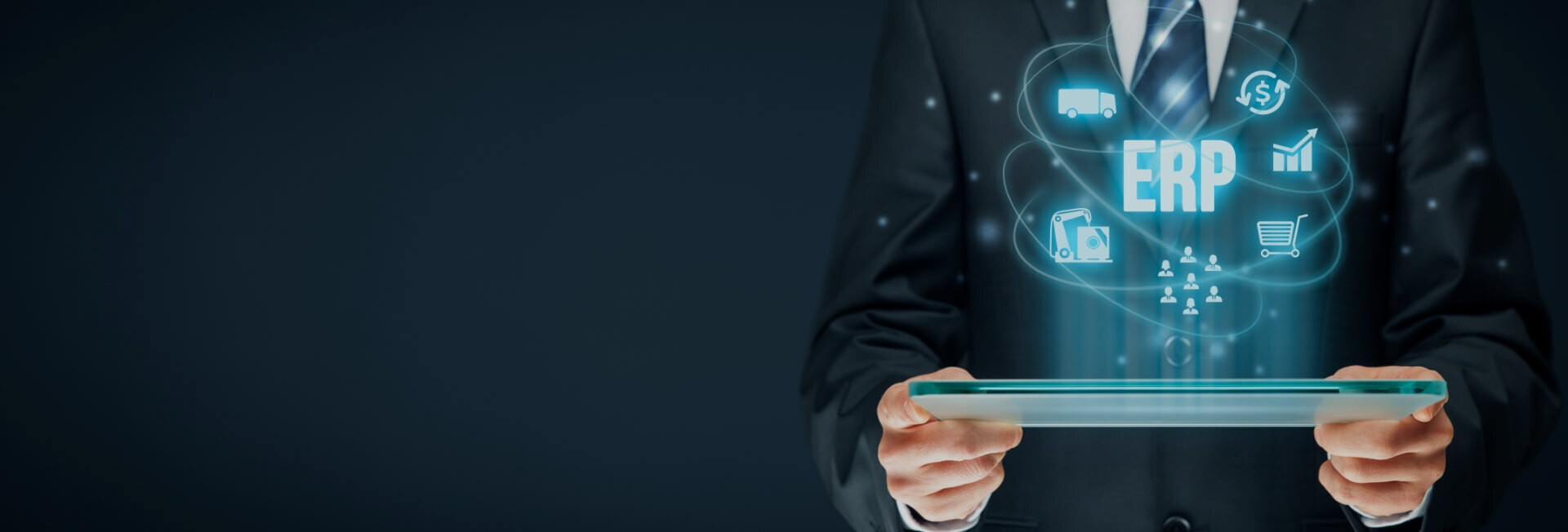
The role of data synchronization in enabling predictive analytics and machine learning
- Home
- Resources
- ERP Integration in Logistics
- The role of data synchronization in enabling predictive analytics and machine learning
The Role of Data Synchronization in Enabling Predictive Analytics and Machine Learning
In today's data-driven world, organizations are constantly looking for ways to gain insights and make data-driven decisions. Predictive analytics and machine learning have emerged as powerful tools to analyze data and predict future outcomes. However, in order to leverage these technologies effectively, organizations need to ensure that their data is accurate, up-to-date, and synchronized across all systems and platforms. This is where data synchronization plays a crucial role.
What is Data Synchronization?
Data synchronization is the process of ensuring that data is consistent and up-to-date across multiple systems or databases. It involves the continuous exchange of data between systems to ensure that the information is accurate and aligned. Data synchronization is essential for organizations that have multiple applications, databases, or systems that need to share and access the same data.
The Importance of Data Synchronization for Predictive Analytics
Predictive analytics relies heavily on accurate and reliable data. The quality of the data used for analysis directly impacts the accuracy and effectiveness of predictive models. By synchronizing data across various systems, organizations can ensure that the data used for predictive analytics is consistent, complete, and up-to-date.
Data synchronization enables organizations to aggregate data from multiple sources and integrate it into a single, unified dataset. This unified dataset provides a holistic view of the organization's data, allowing for more accurate predictions and insights. Without data synchronization, organizations may end up with fragmented and inconsistent data, leading to inaccurate predictions and unreliable insights.
The Role of Data Synchronization in Machine Learning
Machine learning algorithms learn from data to make predictions or take actions. These algorithms require large amounts of high-quality training data to produce accurate and reliable results. Data synchronization plays a vital role in providing the necessary data for training machine learning models.
By synchronizing data across systems, organizations can create a centralized repository of clean, consistent, and up-to-date data for training machine learning models. This ensures that the models are trained on the most relevant and accurate data, leading to better predictions and outcomes.
The Benefits of Data Synchronization for Organizations
Data synchronization offers several benefits for organizations looking to leverage predictive analytics and machine learning. Some of the key benefits include:
1. Improved Data Accuracy and Consistency
By synchronizing data across systems, organizations can ensure that the data used for analysis and modeling is accurate and consistent. This helps in avoiding errors or discrepancies that can lead to incorrect predictions or insights.
2. Enhanced Data Accessibility and Availability
Data synchronization enables real-time updates and ensures that the most up-to-date data is available for analysis and modeling. This allows organizations to make faster and more informed decisions based on the latest information.
3. Streamlined Business Processes
Data synchronization helps in streamlining business processes by integrating data from various sources and systems. This eliminates the need for manual data entry or data migration, saving time and reducing the risk of errors.
4. Improved Predictive Analytics and Machine Learning Models
By providing accurate and consistent data, data synchronization improves the performance and reliability of predictive analytics and machine learning models. This leads to more accurate predictions, better insights, and improved decision-making.
Implementing Data Synchronization for Predictive Analytics and Machine Learning
Implementing data synchronization for predictive analytics and machine learning requires a comprehensive approach. Here are some key steps to consider:
1. Define Data Integration Strategy
Start by defining a data integration strategy that aligns with your organization's goals and requirements. This includes identifying the systems and databases that need to be synchronized, determining the frequency and method of data exchange, and establishing data governance policies.
2. Choose the Right Data Synchronization Solution
There are several data synchronization solutions available in the market. It's important to choose a solution that can handle the complexity and scale of your organization's data. Consider factors such as data volume, data types, real-time updates, and integration capabilities when selecting a data synchronization solution.
3. Integrate Data Synchronization with Existing Systems
Integrating data synchronization with existing systems and workflows is essential for seamless data exchange. This may involve implementing data connectors, APIs, or middleware to facilitate the transfer of data between systems. Work closely with IT teams and solution providers to ensure smooth integration.
4. Monitor and Maintain Data Quality
Data quality is crucial for accurate predictive analytics and machine learning. Implement data quality monitoring tools and processes to identify and address any issues or anomalies in the synchronized data. Regularly audit and validate the data to ensure its integrity and reliability.
Conclusion
Data synchronization plays a critical role in enabling predictive analytics and machine learning. By ensuring that data is accurate, consistent, and up-to-date across systems, organizations can leverage these technologies to gain valuable insights and make data-driven decisions. Implementing data synchronization requires careful planning, the right tools, and a focus on data quality. With the right approach, organizations can unlock the full potential of predictive analytics and machine learning.