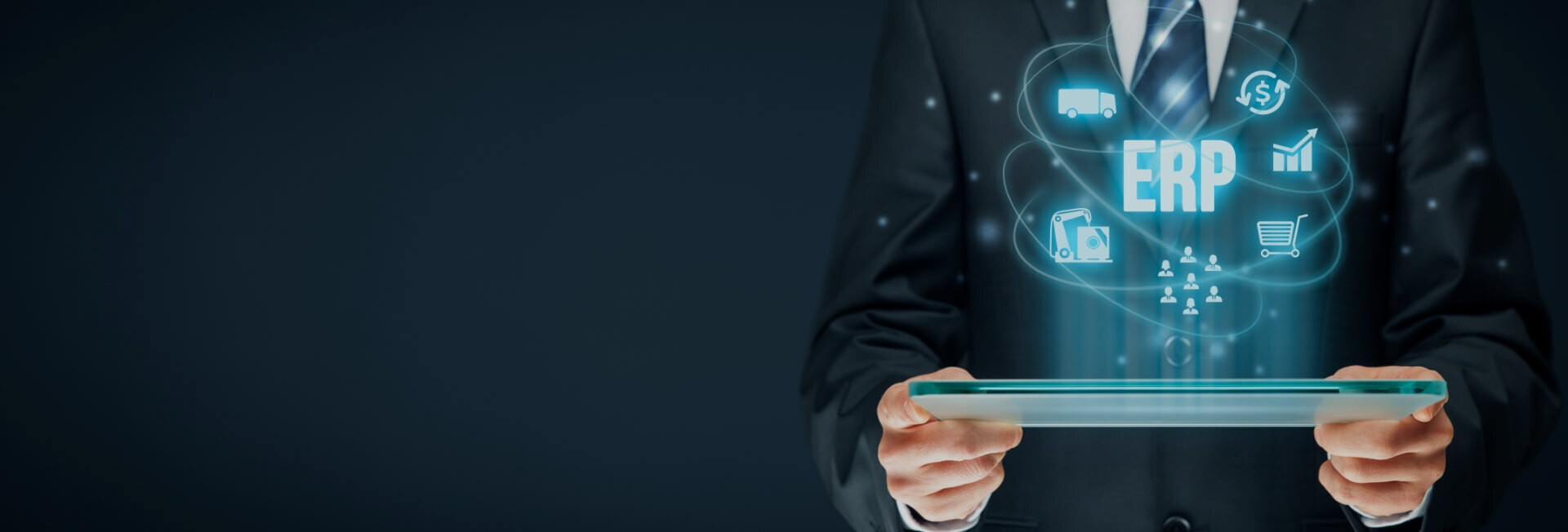
The importance of data quality management in ERP data integration
- Home
- Resources
- ERP Integration in Logistics
- The importance of data quality management in ERP data integration
The Key Role of Data Quality Management in ERP Data Integration
ERP (Enterprise Resource Planning) systems have become an integral part of modern businesses, providing a centralized platform to manage various business processes. From finance and accounting to supply chain and human resources, ERP software streamlines operations and promotes efficiency. However, the success of an ERP implementation heavily relies on the quality of data being integrated into the system. Poor data quality can lead to inaccurate reporting, inefficient workflows, and ultimately, hinder the overall success of the ERP system.
Why Data Quality Management is Crucial in ERP Data Integration
ERP data integration involves consolidating data from multiple sources into a single, unified system. This process requires careful planning and execution to ensure that the data being integrated is accurate, consistent, and reliable. This is where data quality management comes into play.
Data quality management refers to the processes and techniques used to ensure the accuracy, completeness, and consistency of data. It involves identifying and rectifying any errors, inconsistencies, or duplicates in the data before it is integrated into the ERP system. By implementing data quality management practices, businesses can ensure that the data being used for decision-making and analysis is reliable and trustworthy.
The Impact of Poor Data Quality in ERP Data Integration
Poor data quality can have a significant impact on the success of ERP data integration. Here are some of the key consequences of neglecting data quality management:
1. Inaccurate Reporting
One of the primary purposes of an ERP system is to provide accurate and real-time reporting. However, if the data being integrated into the system is of poor quality, the reports generated will be inaccurate and unreliable. This can lead to incorrect decision-making and hinder the ability of the organization to respond effectively to market changes or make informed strategic decisions.
2. Inefficient Workflows
Poor data quality can disrupt workflows and processes within the organization. Inaccurate or incomplete data can result in delays, errors, and inefficiencies in various business processes. For example, if customer data is not properly integrated into the ERP system, it can lead to delays in order processing, incorrect billing, and poor customer service. By ensuring data quality, organizations can optimize their workflows and improve overall operational efficiency.
3. Decreased Customer Satisfaction
Data quality issues can directly impact customer satisfaction. Inaccurate or outdated customer information can lead to poor customer service, missed opportunities, and lost revenue. For example, if a customer's contact information is not updated in the ERP system, the organization may miss out on important communication or fail to address customer needs effectively. By maintaining data quality, organizations can provide better customer service and enhance overall customer satisfaction.
4. Compliance and Legal Risks
Poor data quality can also expose organizations to compliance and legal risks. Inaccurate or incomplete data can lead to non-compliance with regulatory requirements or legal obligations. This can result in legal penalties, reputational damage, and loss of business opportunities. By implementing data quality management practices, organizations can mitigate these risks and ensure compliance with applicable regulations.
The Role of Data Quality Management in ERP Data Integration
Data quality management plays a critical role in ensuring the success of ERP data integration. Here are some of the key benefits and practices of data quality management:
1. Data Cleansing and Standardization
Data cleansing involves identifying and rectifying any errors, inconsistencies, or duplicates in the data. This process ensures that the data being integrated into the ERP system is accurate and reliable. Standardization, on the other hand, involves establishing consistent data formats, conventions, and definitions. By cleansing and standardizing data, organizations can improve data quality and ensure consistency across the ERP system.
2. Data Validation and Verification
Data validation involves checking the accuracy and completeness of data during the integration process. This ensures that only valid and reliable data is being integrated into the ERP system. Verification, on the other hand, involves cross-referencing data with external sources or conducting data audits to ensure its accuracy. By validating and verifying data, organizations can minimize the risks of integrating incorrect or incomplete data into the ERP system.
3. Data Governance and Data Security
Data governance refers to the establishment of policies, processes, and controls to ensure the proper management and use of data. It involves defining data ownership, access rights, and data quality standards. Data security, on the other hand, involves protecting data from unauthorized access, loss, or corruption. By implementing robust data governance and security measures, organizations can ensure the integrity and confidentiality of data within the ERP system.
4. Data Integration Testing
Data integration testing involves validating the accuracy and functionality of data integration processes. It helps identify any issues or errors in the data integration process and ensures that the integrated data meets the required quality standards. By conducting thorough data integration testing, organizations can minimize the risks of data quality issues and ensure the successful integration of data into the ERP system.
Conclusion
Data quality management plays a vital role in the success of ERP data integration. By ensuring the accuracy, completeness, and consistency of data, organizations can optimize their workflows, improve decision-making, and enhance overall operational efficiency. Poor data quality can have significant consequences, including inaccurate reporting, inefficient workflows, decreased customer satisfaction, and compliance risks. Therefore, it is essential for organizations to prioritize data quality management and implement robust practices to ensure the success of their ERP data integration initiatives.