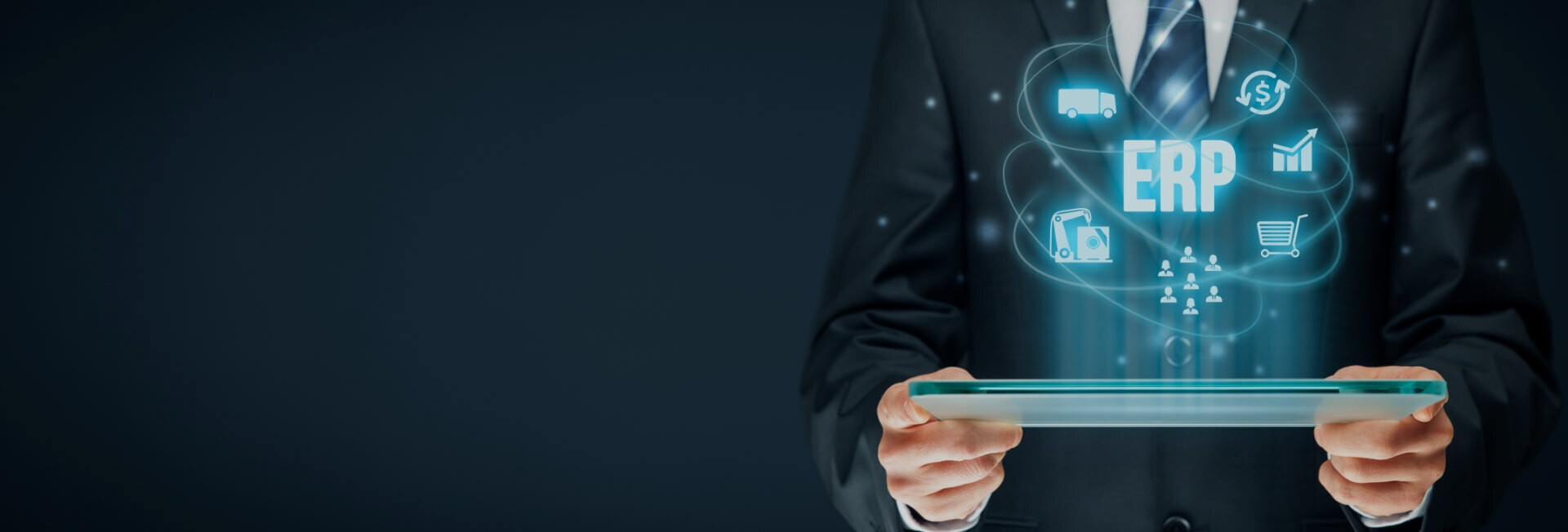
The challenges of integrating data from machine learning algorithms in ERP systems
- Home
- Resources
- ERP Integration in Logistics
- The challenges of integrating data from machine learning algorithms in ERP systems
The Challenges of Integrating Data from Machine Learning Algorithms in ERP Systems
In today's digital world, businesses are constantly seeking ways to optimize their operations and improve efficiency. One of the key ways to achieve this is through the integration of data from machine learning algorithms in Enterprise Resource Planning (ERP) systems. However, this integration process is not without its challenges. In this article, we will explore the obstacles businesses face when integrating data from machine learning algorithms in ERP systems and discuss potential solutions to overcome these challenges.
1. Process Automation and Workflow Optimization
Process automation and workflow optimization are crucial aspects of any ERP system integration. By automating processes and optimizing workflows, businesses can streamline their operations, reduce costs, and increase productivity. However, integrating data from machine learning algorithms into ERP systems can pose challenges in terms of process automation and workflow optimization.
One challenge is the need to align the algorithms with the existing business processes. Machine learning algorithms operate on large datasets and often require custom configurations to fit the specific needs of a business. This can be a complex and time-consuming process, as it involves mapping the algorithms to the existing workflows and ensuring that they are compatible with the ERP system.
Another challenge is the potential disruption to existing workflows. Integrating machine learning algorithms into ERP systems can significantly change how certain processes are executed. This can lead to resistance from employees who are accustomed to the old way of doing things. To overcome this challenge, businesses need to effectively communicate the benefits of the new system and provide adequate training and support to employees during the transition period.
To optimize workflows and ensure seamless integration, businesses should consider working with ERP integration experts who have experience in integrating data from machine learning algorithms. These experts can help businesses identify bottlenecks in their existing workflows and design solutions that leverage the power of machine learning to automate and optimize processes.
2. Data Synchronization and Real-Time Updates
Data synchronization is a critical aspect of ERP system integration. Businesses need to ensure that data from machine learning algorithms is synchronized with the ERP system in real-time to provide accurate and up-to-date information for decision making. However, achieving real-time data updates can be challenging due to various factors.
One challenge is the volume and velocity of data generated by machine learning algorithms. These algorithms often process large amounts of data in real-time, which can put a strain on the ERP system's infrastructure. Businesses need to ensure that their ERP system can handle the increased data load and provide real-time updates without compromising performance.
Another challenge is the need for data standardization and integration. Machine learning algorithms often generate data in different formats and structures, which may not be compatible with the ERP system's data model. In such cases, businesses need to develop data synchronization solutions that can transform and integrate the data from machine learning algorithms into the ERP system seamlessly.
ERP integration services can play a crucial role in addressing these challenges. These services provide expertise in data management and integration, helping businesses develop robust data synchronization solutions. By leveraging their experience and knowledge, ERP integration experts can ensure that data from machine learning algorithms is synchronized with the ERP system in real-time, enabling businesses to make informed decisions based on accurate and up-to-date information.
3. Data Security and Privacy
Data security and privacy are paramount when integrating data from machine learning algorithms in ERP systems. Machine learning algorithms often require access to sensitive data, such as customer information or financial records, to generate meaningful insights. Ensuring the security and privacy of this data is crucial to protect both the business and its customers.
One challenge is the risk of data breaches. Integrating machine learning algorithms into ERP systems increases the attack surface and can make the system more vulnerable to cyber-attacks. Businesses need to implement robust security measures, such as encryption and access controls, to protect the data from unauthorized access.
Another challenge is compliance with data protection regulations. Many countries have strict regulations regarding the collection, storage, and use of personal data. When integrating data from machine learning algorithms into ERP systems, businesses need to ensure that they comply with these regulations to avoid legal and financial consequences.
To address these challenges, businesses should work with ERP integration experts who have expertise in data security and privacy. These experts can help businesses develop and implement security measures that protect sensitive data and ensure compliance with data protection regulations. By prioritizing data security and privacy, businesses can integrate data from machine learning algorithms into ERP systems while minimizing the risk of data breaches and legal issues.
4. ERP Software Implementation and Configuration
ERP software implementation and configuration play a crucial role in successful data integration from machine learning algorithms. Businesses need to ensure that their ERP system is capable of handling the integration and that it is properly configured to accommodate the algorithms and their outputs.
One challenge is the compatibility of the ERP system with the algorithms. Not all ERP systems are designed to integrate seamlessly with machine learning algorithms. Businesses need to evaluate their existing ERP system and determine if it can support the integration. If not, they may need to consider upgrading to a more advanced ERP system or implementing additional software solutions that bridge the gap between the algorithms and the ERP system.
Another challenge is the configuration of the ERP system to accommodate the algorithms' outputs. Machine learning algorithms generate insights and predictions that need to be integrated into the ERP system to provide meaningful information for decision making. This requires configuring the ERP system to accept and process the algorithm outputs in a way that is useful and actionable for the business.
ERP deployment services can provide valuable assistance in addressing these challenges. These services offer expertise in ERP software implementation and configuration, ensuring that the ERP system is properly set up to integrate data from machine learning algorithms. By working with ERP integration experts, businesses can overcome compatibility issues and configure their ERP system to effectively utilize the outputs of the algorithms.
Conclusion
Integrating data from machine learning algorithms in ERP systems offers numerous benefits, including process automation, workflow optimization, and real-time data updates. However, businesses also face challenges in terms of process alignment, data synchronization, data security, and ERP software implementation. By working with ERP integration experts and leveraging their knowledge and experience, businesses can overcome these challenges and successfully integrate data from machine learning algorithms into their ERP systems. This integration can drive efficiency, improve decision making, and ultimately, contribute to the success of the business.