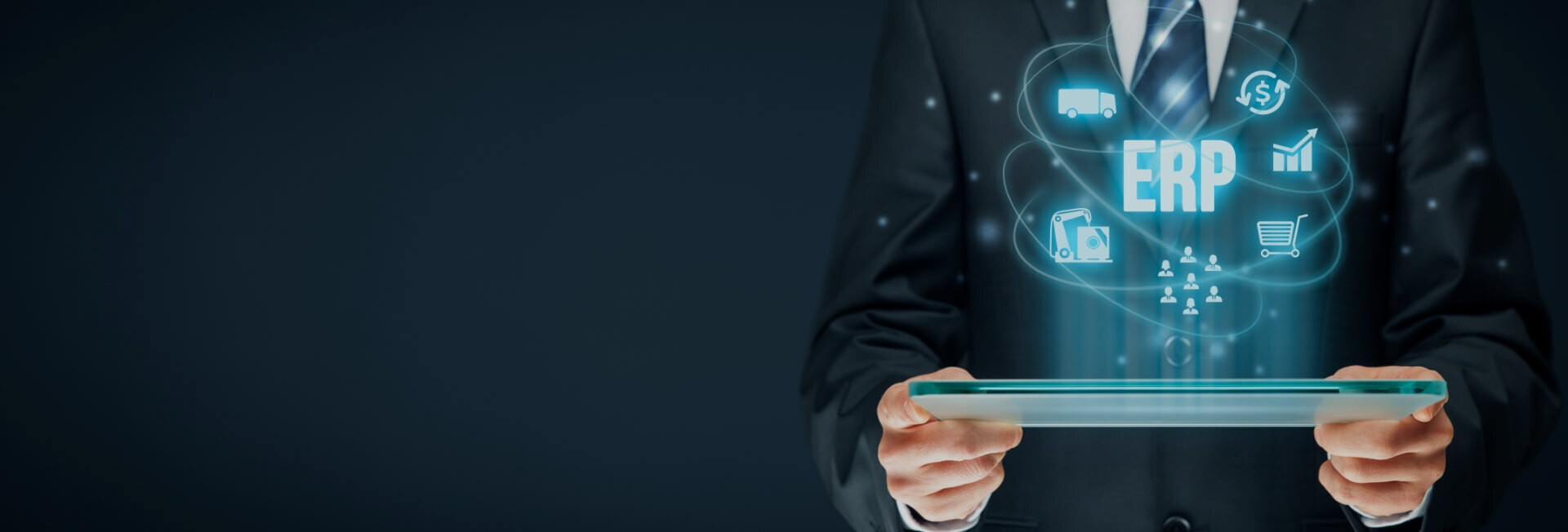
The challenges of data synchronization in data lakes and big data platforms
- Home
- Resources
- ERP Integration in Logistics
- The challenges of data synchronization in data lakes and big data platforms
Introduction
Data lakes and big data platforms have revolutionized the way businesses store and analyze data. They offer the ability to store vast amounts of structured and unstructured data in a centralized repository, enabling organizations to gain valuable insights and make data-driven decisions. However, with the increasing volume and variety of data being generated, one of the major challenges faced by businesses is ensuring data synchronization across these platforms.
The Importance of Data Synchronization
Data synchronization refers to the process of keeping data consistent and up-to-date across different systems, databases, or platforms. In the context of data lakes and big data platforms, it is crucial to have accurate and synchronized data to ensure the reliability and integrity of the analytics and insights generated from the data.
Without proper data synchronization, organizations may encounter various issues such as:
- Inaccurate insights: If the data in the data lake or big data platform is not synchronized with the source systems, the analytics and insights derived from it may be inaccurate or outdated.
- Data inconsistencies: Inconsistent data across different systems can lead to confusion and errors in decision-making. For example, if the sales data in the data lake does not match the sales data in the CRM system, it can result in incorrect sales forecasting and planning.
- Data duplication: Inefficient data synchronization processes can lead to data duplication, where the same data is stored multiple times in different systems. This not only wastes storage space but also increases the risk of data inconsistencies and inaccuracies.
- Data security risks: Inadequate data synchronization can result in security vulnerabilities, as outdated or inconsistent data may not have the necessary security measures in place.
Challenges of Data Synchronization in Data Lakes and Big Data Platforms
Data synchronization in data lakes and big data platforms poses several challenges due to the scale and complexity of the data involved. Some of the key challenges include:
Data Volume and Variety
Data lakes and big data platforms are designed to handle large volumes of data from various sources, including structured, semi-structured, and unstructured data. Synchronizing such diverse data sets can be challenging, as each type of data may have different formats, schemas, and update frequencies. Ensuring the synchronization of data across different data sources requires robust data integration and transformation processes.
Data Velocity
Big data platforms are capable of processing and analyzing data in real-time or near real-time. This means that data synchronization needs to happen quickly and efficiently to ensure that the insights generated from the data are up-to-date. Traditional batch processing methods may not be sufficient to handle the velocity at which data is being generated and updated. Real-time data integration and synchronization solutions are required to keep up with the data velocity.
Data Quality
Data quality is a critical factor in data synchronization. Inaccurate or incomplete data can lead to incorrect insights and decisions. Data cleansing and validation processes need to be implemented to ensure the quality and integrity of the synchronized data. This includes identifying and resolving data inconsistencies, handling missing or erroneous data, and maintaining data integrity throughout the synchronization process.
Data Governance and Security
Data governance and security are important considerations in data synchronization. Organizations need to establish data governance policies and procedures to ensure data privacy, compliance, and security. This includes defining access controls, encryption mechanisms, and auditing processes to monitor and manage data synchronization activities. Compliance with regulations such as GDPR and data protection laws is essential to protect sensitive data during the synchronization process.
Best Practices for Data Synchronization
While data synchronization in data lakes and big data platforms may be challenging, there are several best practices that organizations can follow to overcome these challenges:
1. Data Integration and Transformation
Implement a robust data integration and transformation framework to handle the diverse data sets in the data lake. This includes standardizing data formats, schemas, and data models to ensure consistency across different data sources. Use data integration tools and technologies that support real-time or near real-time data synchronization to keep the data up-to-date.
2. Data Quality Management
Establish data quality management processes and tools to ensure the accuracy and completeness of the synchronized data. This includes data cleansing, validation, and enrichment techniques to address data inconsistencies and errors. Implement data quality monitoring and reporting mechanisms to continuously assess and improve the quality of the synchronized data.
3. Metadata Management
Implement a robust metadata management framework to track and manage the metadata associated with the synchronized data. This includes capturing metadata such as data lineage, data provenance, and data transformation rules. Proper metadata management enables better data discovery, understanding, and governance, ensuring the reliability and traceability of the synchronized data.
4. Data Governance and Security
Establish data governance policies and procedures to ensure data privacy, compliance, and security during the data synchronization process. This includes defining access controls, encryption mechanisms, and auditing processes. Regularly monitor and review data synchronization activities to identify and address any security vulnerabilities or compliance issues.
Conclusion
Data synchronization is a critical aspect of data lakes and big data platforms. It ensures the accuracy, consistency, and integrity of the data, enabling organizations to generate reliable insights and make informed decisions. However, data synchronization in these environments comes with its own set of challenges, including data volume and variety, data velocity, data quality, and data governance. By following best practices and implementing robust data integration, transformation, and quality management processes, organizations can overcome these challenges and achieve effective data synchronization in their data lakes and big data platforms.