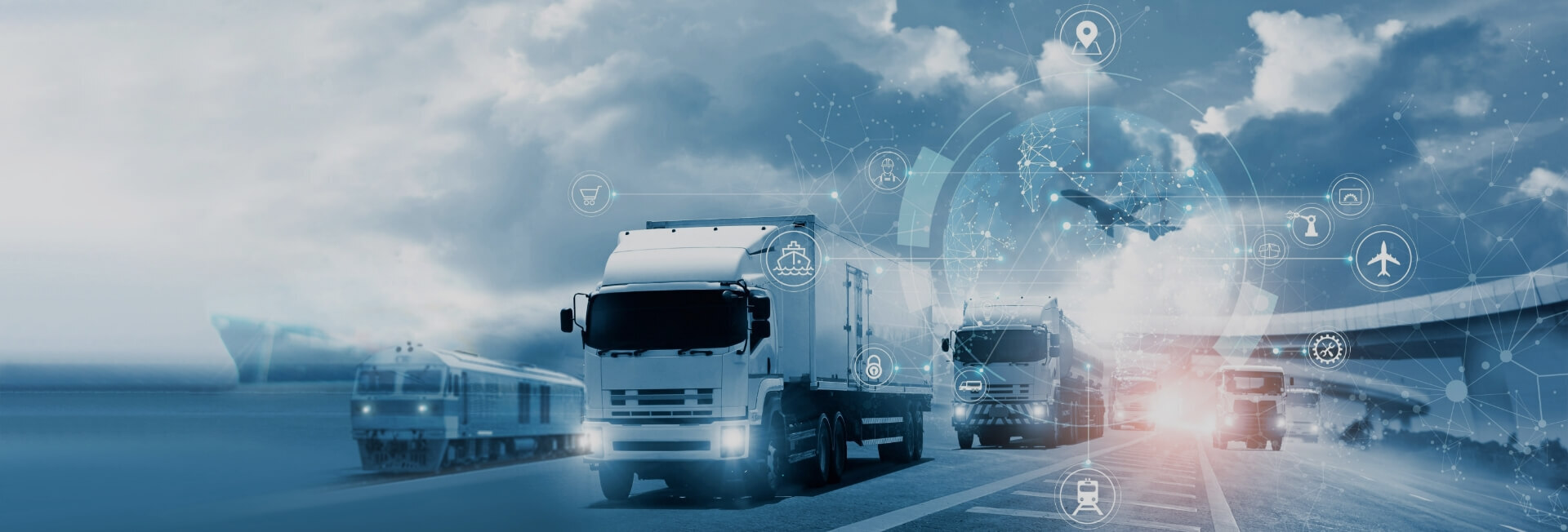
The role of machine learning in fault detection
- Home
- Resources
- CANBus Integration and Application
- The role of machine learning in fault detection
The Power of Machine Learning in Fault Detection
Fault detection is a critical aspect of vehicle diagnostics and telematics. With the advancement in technology, machine learning has emerged as a powerful tool in the automotive industry for predicting and detecting faults in vehicles. In this article, we will explore the role of machine learning in fault detection and how it can revolutionize vehicle telematics and diagnostics.
What is Fault Detection?
Fault detection is the process of identifying abnormal or undesirable behavior in a system. In the context of vehicles, fault detection involves monitoring various parameters and signals to identify potential faults or malfunctions. Traditional fault detection methods rely on predefined thresholds and rules to detect anomalies. However, these methods may not be effective in capturing complex patterns and subtle changes in vehicle behavior.
The Limitations of Traditional Fault Detection Methods
Traditional fault detection methods often rely on predefined thresholds and rules to identify faults. These thresholds and rules are based on expert knowledge and may not capture the full complexity of vehicle behavior. Additionally, these methods may not be able to adapt to new or unknown fault patterns. As vehicles become more complex and interconnected, traditional fault detection methods may struggle to keep up with the increasing complexity.
Furthermore, traditional fault detection methods may generate a high number of false positives and false negatives. False positives occur when the system incorrectly identifies a fault, leading to unnecessary maintenance or repairs. False negatives, on the other hand, occur when the system fails to detect a fault, potentially leading to serious safety risks or costly breakdowns.
The Role of Machine Learning in Fault Detection
Machine learning, a branch of artificial intelligence, offers a promising solution to overcome the limitations of traditional fault detection methods. By leveraging algorithms and statistical models, machine learning can analyze large amounts of data and identify complex patterns and anomalies that may not be apparent to human experts.
One of the key advantages of machine learning in fault detection is its ability to adapt and learn from new data. Instead of relying on predefined thresholds and rules, machine learning algorithms can continuously update their models based on new information. This adaptability allows machine learning algorithms to capture evolving fault patterns and improve detection accuracy over time.
Machine learning can also help reduce false positives and false negatives by considering multiple parameters and signals simultaneously. By analyzing the relationships between different variables, machine learning algorithms can identify subtle changes in vehicle behavior that may indicate a fault. This holistic approach to fault detection can significantly improve the accuracy and reliability of diagnostic systems.
Integration of Machine Learning with Vehicle Telematics
Vehicle telematics, which involves the integration of telecommunications and informatics in vehicles, provides a wealth of data that can be leveraged for fault detection. By combining machine learning algorithms with vehicle telematics, automotive manufacturers and service providers can develop advanced diagnostic systems that can monitor the health of vehicles in real-time.
CANBus communication, a standard protocol used in modern vehicles for interconnecting electronic systems, plays a crucial role in vehicle telematics. By accessing data from various CANBus devices and sensors, machine learning algorithms can analyze the vehicle's behavior and identify potential faults or anomalies.
Machine learning algorithms can also integrate data from other sources, such as GPS data, weather conditions, and historical maintenance records, to provide a holistic view of the vehicle's health. This integration of diverse data sources enables more accurate fault detection and predictive maintenance.
Benefits of Machine Learning in Fault Detection
The integration of machine learning in fault detection offers several benefits for vehicle diagnostics and telematics:
1. Improved Accuracy
Machine learning algorithms can analyze large amounts of data and identify complex patterns, leading to more accurate fault detection. By considering multiple parameters and signals simultaneously, machine learning algorithms can detect subtle changes in vehicle behavior that may indicate a fault.
2. Real-time Monitoring
Machine learning algorithms can continuously monitor vehicle data in real-time, allowing for early detection of faults. This proactive approach to fault detection can prevent costly breakdowns and improve vehicle safety.
3. Predictive Maintenance
By analyzing historical data and identifying patterns, machine learning algorithms can predict potential faults and recommend maintenance actions. This predictive maintenance approach can reduce downtime and maintenance costs.
4. Reduced False Positives and False Negatives
Machine learning algorithms can consider multiple parameters and signals, reducing the number of false positives and false negatives. This leads to more reliable fault detection and reduces unnecessary maintenance or repairs.
Challenges and Considerations
While machine learning offers significant potential in fault detection, there are several challenges and considerations that need to be addressed:
1. Data Quality and Availability
The accuracy and reliability of machine learning algorithms heavily depend on the quality and availability of data. Inaccurate or incomplete data can lead to inaccurate predictions and faulty fault detection. It is crucial to ensure that the data used for training and testing machine learning models is of high quality and representative of real-world scenarios.
2. Interpretability and Explainability
Machine learning algorithms can sometimes be considered as black boxes, making it difficult to interpret and explain their decisions. In safety-critical systems such as automotive diagnostics, it is essential to have transparent and explainable models to gain trust and acceptance from stakeholders.
3. Overfitting and Generalization
Machine learning models may suffer from overfitting, where they perform well on the training data but fail to generalize to new, unseen data. It is crucial to carefully design and validate machine learning models to ensure they can generalize to real-world situations and adapt to evolving fault patterns.
4. Ethical Considerations
Machine learning algorithms should be developed and deployed in an ethical manner, taking into account privacy and security concerns. The collection and use of vehicle data should comply with applicable regulations and ensure the protection of user privacy.
The Future of Fault Detection
The integration of machine learning in fault detection is still in its early stages, but the potential is immense. As vehicles become increasingly connected and autonomous, the need for advanced fault detection systems will continue to grow. Machine learning, combined with vehicle telematics and data integration, can revolutionize vehicle diagnostics and maintenance.
Future advancements in machine learning algorithms, data collection, and processing capabilities will further enhance the accuracy and effectiveness of fault detection systems. With the ability to analyze vast amounts of data in real-time, machine learning algorithms can enable predictive maintenance, reduce downtime, and improve overall vehicle safety.
Conclusion
Machine learning has the power to revolutionize fault detection in the automotive industry. By leveraging algorithms and statistical models, machine learning can analyze large amounts of data, identify complex patterns, and improve the accuracy and reliability of diagnostic systems. The integration of machine learning with vehicle telematics enables real-time monitoring and predictive maintenance, leading to improved vehicle safety and reduced maintenance costs. While there are challenges to overcome, the future of fault detection looks promising with the advancements in machine learning technology.