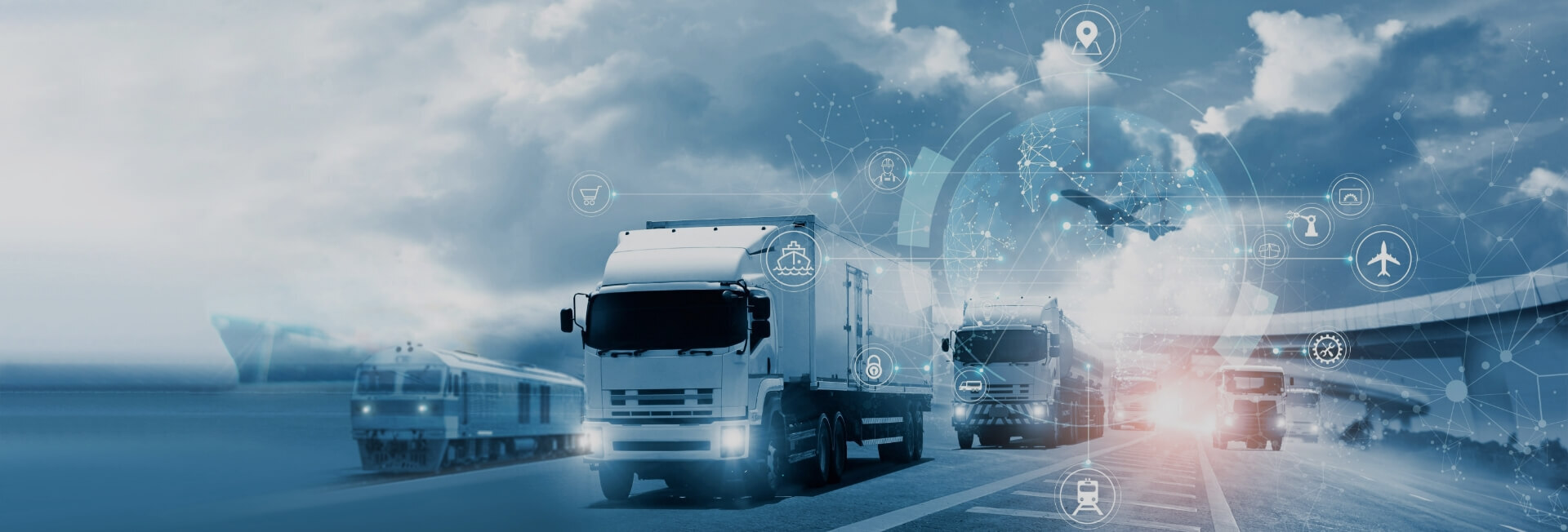
The benefits of using machine learning for fault detection
- Home
- Resources
- CANBus Integration and Application
- The benefits of using machine learning for fault detection
The Power of Machine Learning for Fault Detection
In today's world, where cars are becoming increasingly advanced and complex, ensuring their proper functioning and detecting faults in a timely manner is of utmost importance. Traditional methods of fault detection and diagnosis often fall short when it comes to handling the vast amount of data generated by modern vehicles. This is where machine learning comes to the rescue. By leveraging the power of artificial intelligence and advanced algorithms, machine learning techniques enable efficient and accurate fault detection in vehicles. In this article, we will explore the benefits of using machine learning for fault detection.
1. Improved Vehicle Diagnostics
Machine learning algorithms have the ability to analyze large volumes of data and identify patterns that may not be easily detectable by humans. When applied to vehicle diagnostics, machine learning can effectively identify and diagnose faults in various components of a vehicle, including the engine, transmission, brakes, and more. By analyzing data from sensors and other sources, machine learning algorithms can detect anomalies and patterns indicative of potential faults, allowing for timely repairs and maintenance.
One of the key advantages of machine learning-based vehicle diagnostics is its ability to adapt and learn from real-time data. As the algorithms are exposed to more data, they become increasingly accurate in identifying faults and predicting potential failures. This adaptive nature of machine learning algorithms ensures that the diagnostics process remains up-to-date and effective even as vehicles and their components evolve over time.
2. Enhanced Fault Detection in Telematics Systems
Telematics systems, which combine telecommunications and informatics in vehicles, generate vast amounts of data related to vehicle performance, location, and other parameters. By integrating machine learning algorithms into telematics systems, it becomes possible to detect faults and anomalies in real-time, allowing for proactive maintenance and minimizing vehicle downtime.
Machine learning algorithms can analyze telematics data and identify patterns that indicate potential faults or malfunctions. For example, abnormal engine temperature readings or sudden drops in fuel efficiency could be indicative of an underlying issue. By continuously monitoring and analyzing telematics data, machine learning algorithms can detect these patterns and alert fleet managers or maintenance personnel, enabling them to take prompt action.
3. Efficient Fault Detection with CANBus Communication
CANBus (Controller Area Network Bus) communication is a standard protocol used in modern vehicles for interconnecting various electronic control units (ECUs) and sensors. By integrating machine learning algorithms with CANBus communication, it is possible to perform fault detection and diagnosis in a highly efficient manner.
Machine learning algorithms can analyze the data exchanged over the CANBus network and identify patterns indicative of faults or anomalies. For example, an abnormal spike in engine RPM or an unexpected drop in vehicle speed could be signs of a potential issue. By continuously monitoring the CANBus data and applying machine learning algorithms, faults can be detected and diagnosed in real-time, allowing for timely repairs and maintenance.
4. Remote Vehicle Diagnostics and Monitoring
Machine learning techniques can also be leveraged for remote vehicle diagnostics and monitoring. By integrating machine learning algorithms with remote monitoring systems, it becomes possible to analyze vehicle data remotely and detect faults without the need for physical access to the vehicle.
Remote vehicle diagnostics systems equipped with machine learning algorithms can analyze data from various sources, such as telematics systems, onboard sensors, and even external sources like weather data. By continuously monitoring this data, machine learning algorithms can detect patterns indicative of faults or potential failures, allowing for proactive maintenance and minimizing vehicle downtime.
Conclusion
Machine learning is revolutionizing the field of fault detection in vehicles. By leveraging the power of artificial intelligence and advanced algorithms, machine learning techniques enable efficient and accurate fault detection, leading to improved vehicle diagnostics, enhanced fault detection in telematics systems, efficient fault detection with CANBus communication, and remote vehicle diagnostics and monitoring. With the ability to analyze large volumes of data and identify patterns that may not be easily detectable by humans, machine learning is transforming the way we detect and diagnose faults in vehicles, ensuring their proper functioning and minimizing downtime.