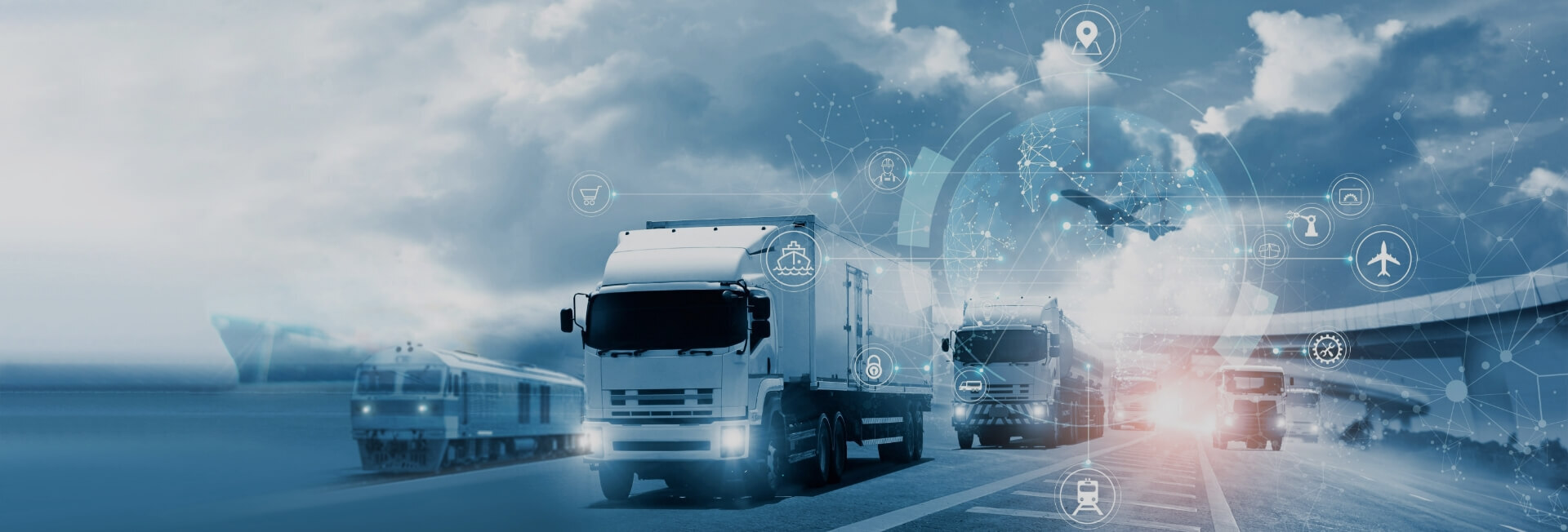
Data integration in automotive predictive analytics
- Home
- Resources
- CANBus Integration and Application
- Data integration in automotive predictive analytics
Data Integration in Automotive Predictive Analytics
In today's digital age, vehicles are becoming increasingly connected and data-driven. With the advent of vehicle telematics and advanced vehicle diagnostic systems, automotive companies are able to collect and analyze vast amounts of data to improve vehicle performance, optimize maintenance schedules, and enhance overall customer experience. However, the real value lies in the ability to integrate and make sense of this data. In this article, we will explore the importance of data integration in automotive predictive analytics and how it enables vehicle diagnostics, fault detection, and remote vehicle diagnostics.
What is Data Integration?
Data integration refers to the process of combining data from various sources and formats into a unified view. In the context of automotive predictive analytics, it involves collecting data from different vehicle systems, such as the engine, brakes, transmission, and sensors, and integrating it into a centralized platform for analysis. This integration allows automotive companies to gain a holistic view of the vehicle's health and performance, enabling them to make informed decisions and take proactive actions.
Vehicle Diagnostics and Fault Detection
One of the primary applications of data integration in automotive predictive analytics is vehicle diagnostics and fault detection. By collecting data from various sensors and systems within the vehicle, automotive companies can monitor the health and performance of different components in real-time. This data can then be analyzed to identify potential faults or anomalies, allowing for early detection and preventive maintenance.
For example, if a vehicle's engine temperature is consistently higher than normal, it could indicate a potential issue with the cooling system. By integrating data from the engine sensors, temperature sensors, and other relevant systems, automotive companies can detect this anomaly and alert the driver or initiate a maintenance request. This proactive approach not only prevents breakdowns and costly repairs but also improves overall vehicle reliability and customer satisfaction.
CANBus Communication and Integration
A key component of data integration in automotive predictive analytics is the Controller Area Network (CANBus). The CANBus is a standardized communication protocol used in modern vehicles to enable communication between various electronic control units (ECUs). It allows for the exchange of data and commands between different vehicle systems, such as the engine, transmission, ABS, and infotainment system.
By integrating data from the CANBus, automotive companies can access a wealth of information about the vehicle's performance, including engine RPM, vehicle speed, fuel consumption, and more. This data can be used to monitor the vehicle's health, analyze driving patterns, and identify potential issues. Additionally, the CANBus enables the integration of third-party devices and solutions, providing even more insights and capabilities for automotive predictive analytics.
Fleet Data Integration and Management
For fleet operators, data integration is particularly crucial for managing and optimizing a large number of vehicles. By integrating data from multiple vehicles in a fleet, automotive companies can gain insights into the overall fleet health, identify trends and patterns, and optimize maintenance schedules.
For example, by analyzing data from multiple vehicles, fleet operators can identify common issues or failure patterns across the fleet. This information can be used to proactively address these issues and prevent similar failures in other vehicles. Additionally, data integration enables fleet-wide reporting and analytics, allowing for better decision-making and resource allocation.
Remote Vehicle Diagnostics
Another important application of data integration in automotive predictive analytics is remote vehicle diagnostics. By integrating data from connected vehicles, automotive companies can remotely monitor and diagnose vehicles without the need for physical inspection. This not only saves time and resources but also enables proactive maintenance and support.
For example, if a vehicle's check engine light turns on, automotive companies can remotely access the vehicle's diagnostic data and analyze the issue. Based on this analysis, they can provide recommendations to the driver, such as scheduling a maintenance appointment or taking immediate action. This remote diagnostics capability improves the overall efficiency of the maintenance process and reduces downtime for the driver.
Conclusion
Data integration is a critical component of automotive predictive analytics, enabling vehicle diagnostics, fault detection, and remote vehicle diagnostics. By integrating data from various vehicle systems and sources, automotive companies can gain a holistic view of the vehicle's health and performance, allowing them to make informed decisions and take proactive actions. Whether it's monitoring the health of a single vehicle or managing a fleet of vehicles, data integration plays a crucial role in optimizing maintenance schedules, improving reliability, and enhancing overall customer experience.