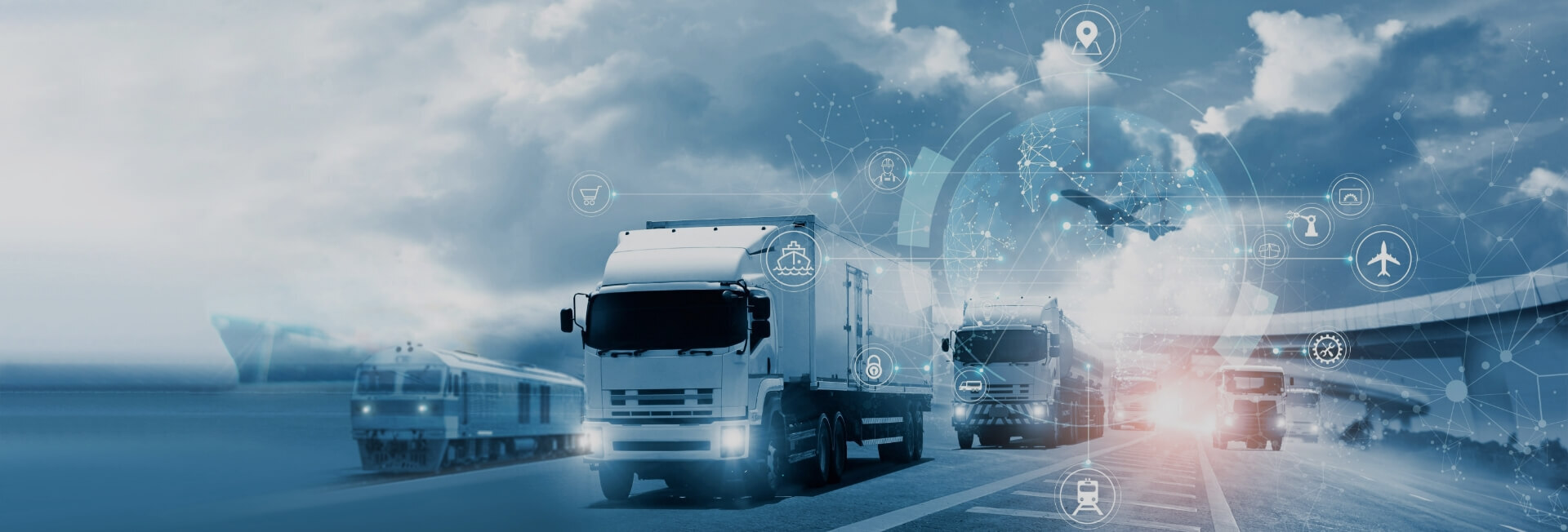
Data integration challenges in automotive big data management
- Home
- Resources
- CANBus Integration and Application
- Data integration challenges in automotive big data management
The Importance of Data Integration in Automotive Big Data Management
In today's digital age, the automotive industry is generating an enormous amount of data from various sources, including vehicle diagnostics, telematics, and fault detection systems. This data is crucial for vehicle manufacturers, fleet operators, and service centers to monitor vehicle health, improve performance, and provide remote diagnostics. However, managing and integrating this vast amount of data can be a significant challenge.
Understanding CANBus Communication
One of the key challenges in automotive big data management is the integration of data from the CANBus (Controller Area Network) communication system. The CANBus is a network of interconnected devices and sensors in a vehicle that communicate with each other to monitor and control various functions. It is responsible for collecting data related to engine performance, fuel efficiency, vehicle speed, and more.
The integration of data from the CANBus is crucial for vehicle diagnostics, remote monitoring, and fault detection. However, the diverse range of canbus devices and protocols used by different vehicle manufacturers makes data integration a complex task. Each vehicle manufacturer may have its own canbus solutions and interfaces, making it challenging to develop a standardized approach for data integration.
The Complexities of Automotive Data Integration
Automotive data integration involves collecting, aggregating, and analyzing data from various sources, including vehicle sensors, telematics systems, and external data sources. The integration process involves mapping and transforming data from different formats and systems to ensure compatibility and consistency.
One of the primary challenges in automotive data integration is the lack of standardized data formats and protocols. Different vehicle manufacturers may use different data formats and communication protocols, making it difficult to integrate data from multiple sources. This requires the development of custom integration solutions and interfaces to ensure seamless data flow.
Another challenge is the volume and velocity of data generated by vehicles. With the increasing number of connected vehicles and the growing complexity of vehicle systems, the amount of data generated is skyrocketing. Managing and processing this vast amount of data in real-time requires robust data integration solutions that can handle high volumes of data and ensure timely delivery.
Overcoming Data Integration Challenges
To overcome the data integration challenges in automotive big data management, several solutions and strategies can be implemented:
1. Standardization of Data Formats and Protocols
Standardizing data formats and protocols across different vehicle manufacturers can simplify the integration process. This would allow for the development of standardized integration solutions and interfaces that can be used across the industry. The adoption of industry-wide standards would enable seamless data integration and improve interoperability between different systems.
2. Development of CANBus Interface and Integration Tools
The development of CANBus interface and integration tools specifically designed for automotive data integration can simplify the process. These tools can provide a unified interface for connecting and integrating data from various canbus devices and protocols. They can also automate the mapping and transformation of data, reducing manual effort and ensuring data consistency.
3. Implementation of Real-time Data Processing
Real-time data processing is crucial for automotive data integration, especially in applications such as vehicle health monitoring and remote diagnostics. Implementing stream processing technologies can enable the processing and analysis of data in real-time, allowing for timely detection of faults and proactive maintenance. This requires the use of high-performance data integration platforms that can handle the velocity and volume of data generated by vehicles.
4. Adoption of Cloud-based Data Integration Solutions
Cloud-based data integration solutions can provide scalability and flexibility for automotive big data management. By leveraging cloud computing resources, automotive companies can handle large volumes of data and scale their data integration infrastructure as needed. Cloud-based solutions also enable data sharing and collaboration between different stakeholders, improving overall data management and analysis capabilities.
Conclusion
Data integration is a critical aspect of automotive big data management. The integration of data from various sources, including vehicle diagnostics, telematics, and fault detection systems, is essential for vehicle health monitoring, remote diagnostics, and performance improvement. However, the diverse range of canbus devices and protocols used by different vehicle manufacturers poses significant challenges in data integration.
To overcome these challenges, standardization of data formats and protocols, the development of canbus interface and integration tools, implementation of real-time data processing, and adoption of cloud-based data integration solutions can be effective strategies. By addressing these challenges and implementing robust data integration solutions, the automotive industry can harness the power of big data to drive innovation and improve vehicle performance.